Factors Blog
Insights Across All Things B2B Account Intelligence & Analytics

The Complete Guide to Building A SaaS CMO Dashboard
Tracking campaign performance across marketing channels can be a tedious and unintuitive process. CMOs often have to rely on individual department reports to gather a summary of how the team is performing – an endeavor that leaves many gaps and relies heavily on guesswork.
In addition to the time it takes to compile reports, derive actionable insights, and chart next-steps, the traditional way of doing things is prone to several manual errors.
The solution? The perfect CMO dashboard that helps streamline your work based on relevant analytics and metrics under one roof.
This article shows how powerful CMO dashboards can be used to drive data-driven business decisions.
TL;DR
- CMO dashboards offer a holistic view of customer data-related metrics and KPIs through intuitive visualizations.
- The benefits of SaaS companies using CMO dashboards include rapid decision-making, sensible use of time and resources, improved ROI, and unified visibility of marketing performance.
- Before you build a comprehensive CMO dashboard, pay close attention to user-friendly design, use helpful visualizations, integrate platforms to consolidate data, and measure the right metrics/KPIs.
- SaaS businesses must track metrics/KPIs such as conversions, leads, traffic, conversion rates, acquisition cost per channel, retention and churn rates, customer lifetime value, and revenue and pipeline velocity by channel source. A CMO dashboard helps visualize these metrics and KPIs with clarity.
What is a CMO dashboard?
A CMO dashboard is a tool that reports and visualizes KPIs and metrics related to a company's marketing activities across demand gen, content, paid, events, and more. It is a centralized, bird’s eye view that helps marketing executives monitor and analyze marketing initiatives and performance in real-time.
There is no hard and fast rule to building a CMO dashboard for your SaaS company. That being said, there are certain elements that are essential for marketing leaders to identify patterns, track progression, and optimize strategies to drive bottom-line business objectives.
We’ll explore these essential ingredients in later sections of this blog.
Why do CMOs need to use dashboards?
Here’s a look at the top benefits of using a well-designed CMO dashboard:
1. Improves decision-making
When making expensive business decisions, relying on guesswork can cost you heavily. CMO dashboards help marketing leaders make well-informed and accelerated decisions based on data-driven insights.
For example, a CMO dashboard can help you identify which channels and marketing campaigns are not performing if you're making budget-related decisions. It also enables you to identify the reason behind poor campaign performance and the following steps to be taken.
Let's say you have the company blog, paid advertising, and podcasts as key marketing channels. A deep dive into the dashboard will help you uncover how much revenue each channel can generate, the cost of leads from each touchpoint, and how long it typically takes them to convert.

2. Offers a quick overview of data without wasting time and resources
A CMO dashboard lets you save hours of work and wasted resources on collecting and processing raw data from scratch.
CMO dashboards extract and present an accurate overview of essential data from platforms or sources such as LinkedIn, Facebook, YouTube, articles, Google Ads, and more.
For example, let’s say you’re calculating the number of sessions on your website that come through various channels. A CMO dashboard will gather the data from all the channels, creating an easy visual for you to see which has driven the most sessions.

3. Helps identify trends and patterns
With all the data condensed onto one dashboard, CMOs and their teams can collectively take note of repetitive patterns in their target audience. This helps them further understand which type of campaigns work well on what channel and when leading to the ability to adapt campaign resources on the go.
For example, the company publishes a blog on the website every Tuesday. But they also post a video on LinkedIn on the same day. Over time, a CMO dashboard can display which content formats perform better on Tuesdays, helping the team double down their focus on that channel alone.
Key considerations when building a CMO Dashboard
An effective CMO dashboard is the central hub for monitoring marketing performance, aligning teams, and driving data-backed decisions. When designed thoughtfully, it becomes an invaluable strategic asset.
1. Intuitive and action-oriented design
A CMO dashboard is defined by its ease of use and ability to drive decisions. A cluttered, text-heavy layout overwhelms users with data but does not provide enough context to make decisions.
In contrast, a clean, visual interface tells a straightforward performance story that connects insights to actions and helps make data-backed decisions. Here are a few considerations for an insightful dashboard.
Prioritize key metrics

Prioritize the most critical metrics and add impactful visualizations like charts, graphs, gauges, and indicators to showcase them. For instance, use:
- Line charts to display trends over time for metrics like web traffic, conversions, etc.
- Comparison charts or pie charts to show channel effectiveness, campaign ROI, and more
- Funnel visualization to showcase drop-offs across the customer journey
- Alerts and gauges to highlight metrics nearing goals or thresholds
Optimize Information Absorption
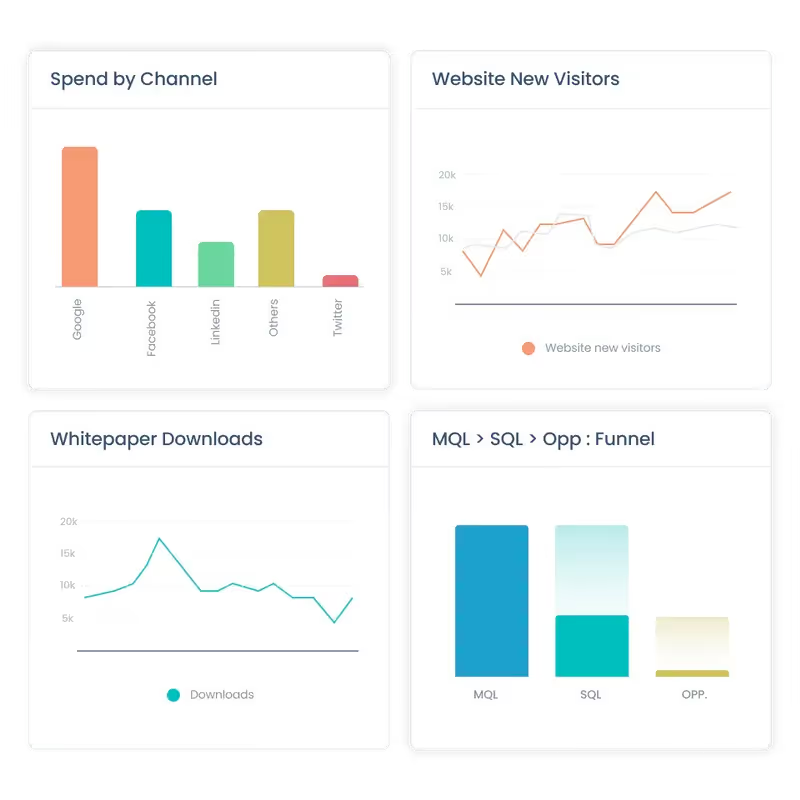
Use color coding, alerts, and trends to highlight priority areas at a glance. Make sure that your dashboards have ample white space for better data absorption.
Also, include explanatory captions and annotations to provide essential context.
Offer Intuitive Interactions
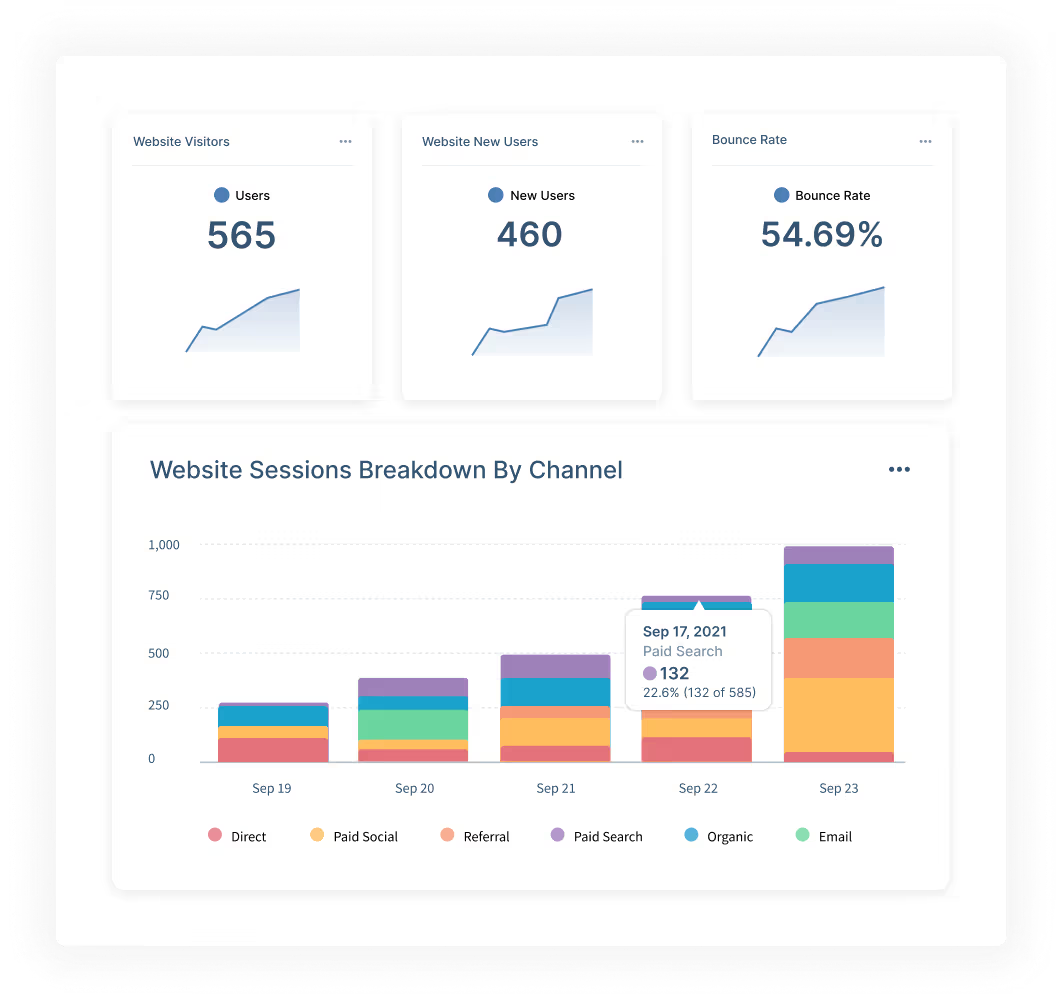
Offer intuitive interactions like drill-downs, filtering, and segmenting to customize views on demand. For instance, help users:
- Filter data by categories, campaigns, regions, or date ranges
- Drill down from aggregate metrics to detailed data breakdowns
- Isolate underperforming categories and campaigns
Such flexibility allows slicing and dicing data along different dimensions for deeper analysis.
Streamline Navigation
Create tabs or dropdowns to switch between views, campaigns, time frames, and other dimensions. Optimize compatibility across devices so users can access the dashboard anywhere conveniently.
Most importantly, align terminologies and visualizations to thought processes familiar to users through day-to-day work. Simplicity and intuitiveness accelerate adoption.
2. Unified Data Foundation Across Teams

Marketing cannot drive impact alone. Close alignment across teams provides comprehensive visibility into the end-to-end customer journey.
Break Departmental Silos
Integrate your CMO dashboard tightly with essential systems like CRM, sales analytics, web analytics, finance systems, etc. Automatically sync campaign data, lead status changes, deal progress, and other cross-functional data flows.
For instance, pull web visitor profiles from analytics platforms to enrich lead records. Or, pass lead quality assessment and scoring metrics from marketing to sales for better follow-ups.
Shared Visibility Through Attribution
Compare the performance of marketing-generated vs. sales-generated pipelines on metrics like lead quality, sales cycle times, win rates, and deal sizes.
Conduct multi-touch attribution to understand marketing's influence at each buying stage. Such unified visibility bridges departmental silos with shared goals and metrics, steering coordinated priorities across teams.
3. Flexibility to Highlight Strategic Focus Areas
While cross-functional data consolidation provides tremendous value, a one-size-fits-all dashboard rarely meets specialized business needs fully. You need the dashboard to be customizable and dynamic.
Customizable Templates
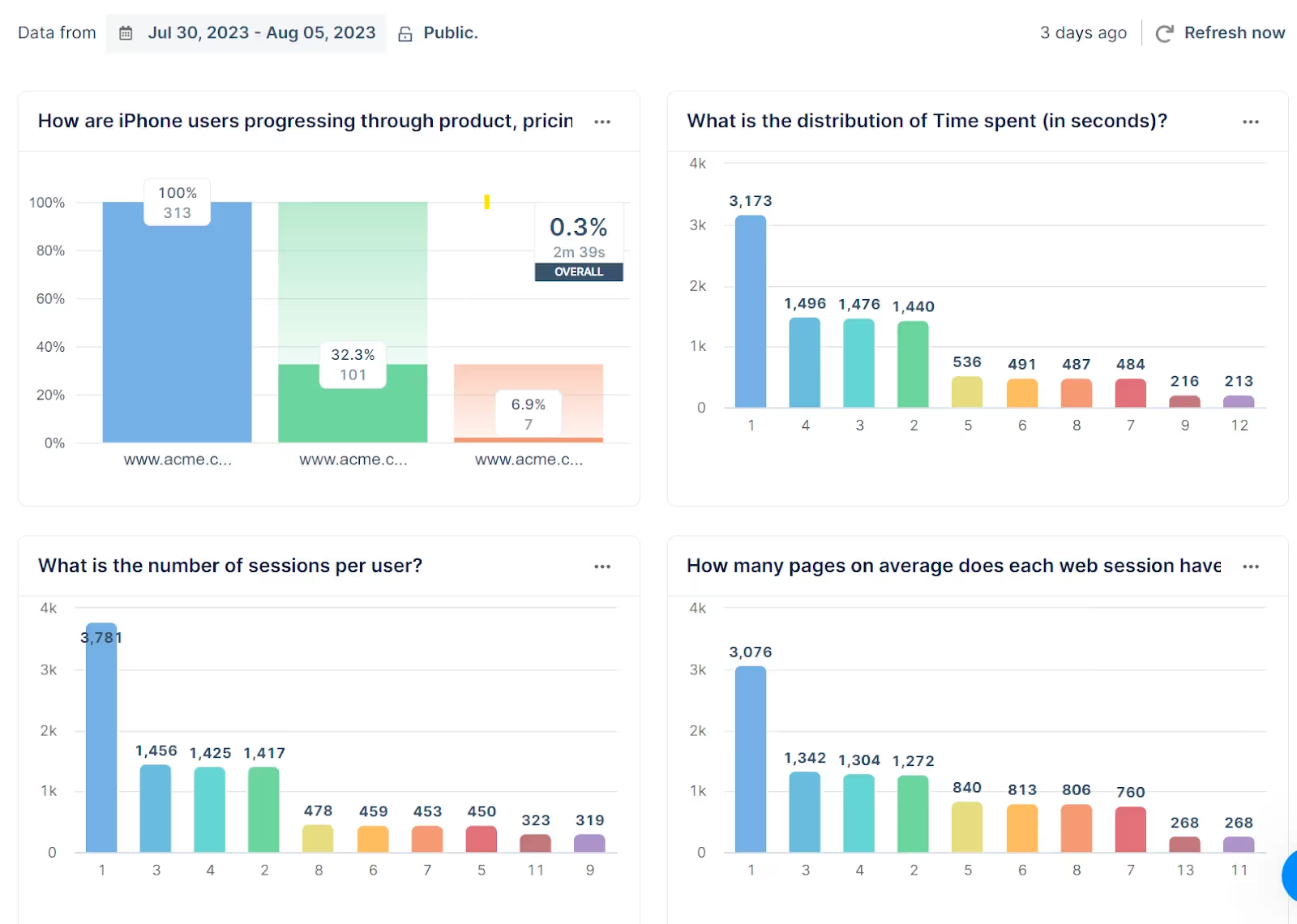
Empower users to highlight metrics aligned to their goals—broad awareness or targeted account-based sales—without relying on IT.
Provide pre-built templates for different personas and use cases that showcase industry-specific benchmarks more relevant to their context.
Dynamic Filtering and Segmentation
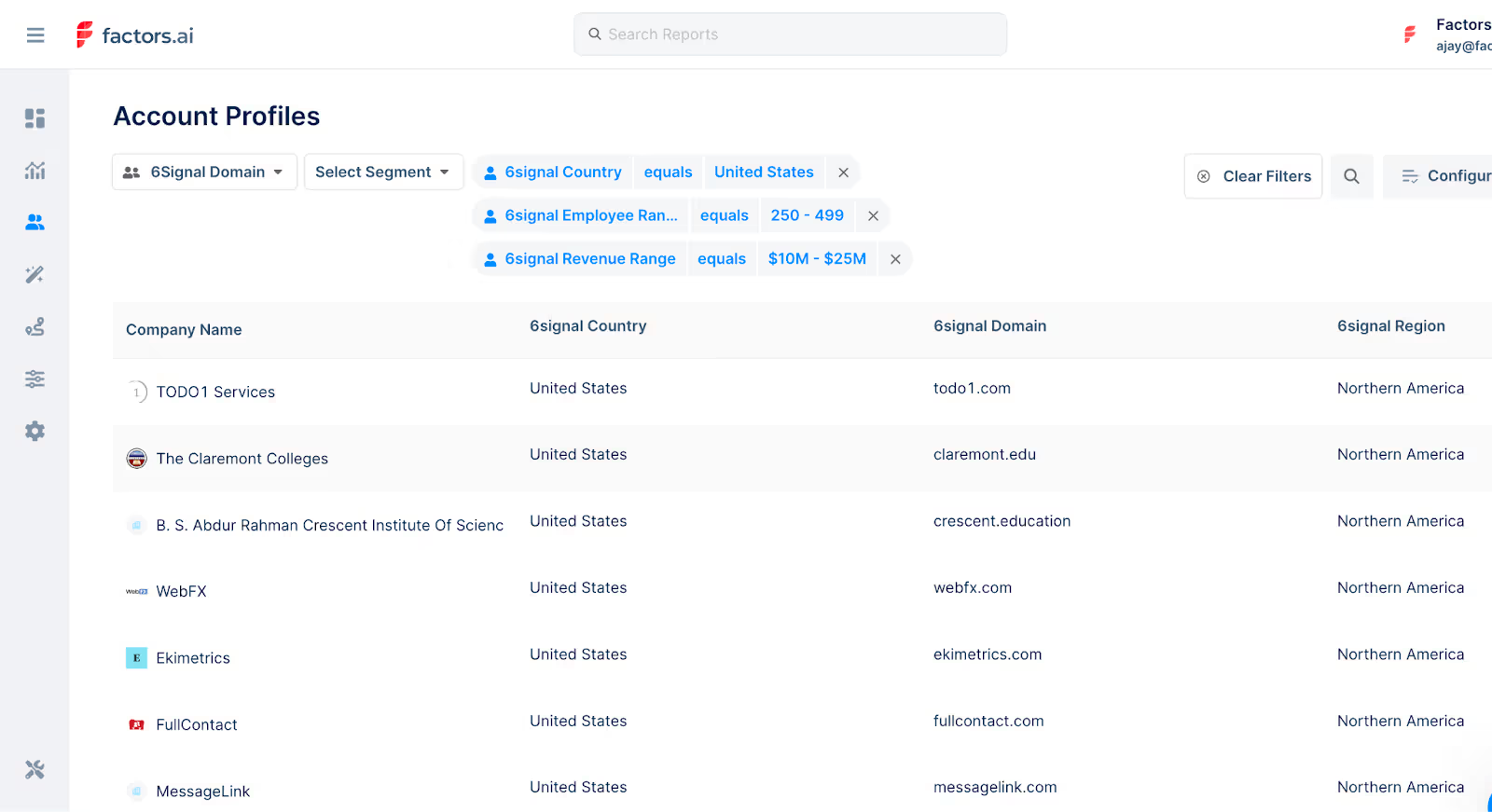
Offer easy-to-use customization options such as an intuitive template builder interface. Let marketers filter data views dynamically by parameters like region, customer persona, product line, etc.
Such flexibility allows teams to analyze performance through different lenses—whether for the leadership team or campaign managers. As business objectives evolve, custom-tailored dashboards stay focused on what matters most.
4. Ongoing Optimization and Iteration
No dashboard gets designed perfectly right from the beginning. As marketing campaigns and strategies adapt in response to market dynamics, your dashboards also continuously realign to maintain relevance.
User Testing and Feedback Analysis
Monitor usage patterns and user feedback to identify navigation, metric, or visualization enhancements: track clicks, hovers, and other interactions to uncover usability issues. Conduct user surveys and interviews to gather feedback.
Maintain Flexibility
Maintain flexibility for adding new datasets from emerging sources and channels. Continually experiment, test, and optimize to fulfill ever-changing information needs most effectively.
Through an intuitive and action-oriented design backed by an integrated, flexible data foundation, the CMO dashboard becomes a powerful nerve center driving shared visibility, coordinated execution, and data-informed decisions across the business.
CMO Dashboard KPIs and Metrics
A CMO dashboard can include tons of KPIs and metrics depending on what you’re trying to track. However, below are the ones that are of topmost priority:
1. Top-of-the-funnel metrics such as engagement, traffic, leads
Top-of-the-funnel metrics are brand awareness metrics like the engagement rate, traffic, and leads generated. Most SaaS marketing executives track these to attract the target audience and turn them into long-term subscribers.
1. Engagement rates are metrics used to measure and track the active involvement of your target audience for the content you produce. The engagement rate formula is:
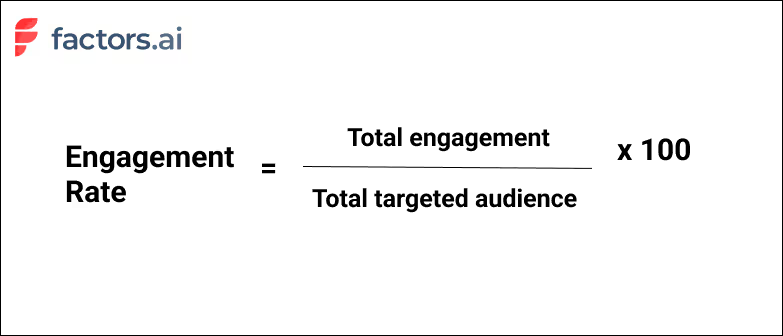
2. Traffic for SaaS companies is the volume or the total number of visitors their website gets over a certain time period.
3. Leads generated is a mandatory sales KPI every SaaS company must track. Measuring leads lets you uncover issues and nurture prospects further.
Why do these metrics matter?
Top-of-the-funnel metrics help measure the overall exposure of the target audience to your marketing efforts. Tracking and measuring them gives insight into what can help minimize roadblocks that stop prospects from signing up.
2. Conversion rates and efficiency
1. Conversion rates: measure the number of targeted users who converted from unknown prospects to engaged contacts. The higher the conversion rate, the more impact your marketing strategies have. The formula to calculate the conversion rate is:

2. Efficiency: In marketing, the ROI measures the overall performance of your marketing efforts, and conversion rates directly indicate the performance. That means increasing conversion rates while reducing acquisition costs promises a better ROI and shows better strategy efficiency. The formula to calculate marketing efficiency is:
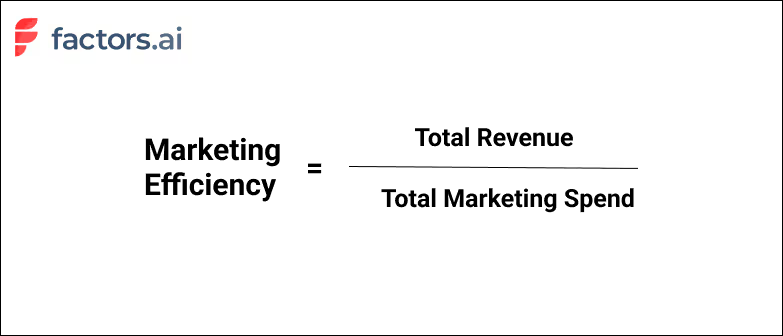
Why do these metrics matter?
Conversion rates and efficiency directly reflect how well your SaaS marketing activities are executed and determine the overall effectiveness of your business campaigns.
3. Cost of acquisition by channel
It’s best to track certain KPIs by marketing channels, such as social media, emails, ads, organic search, etc. Calculating the cost of customer acquisition (CAC) for each channel is one such KPI that indicates the marketing expenses spent on obtaining customers.The formula to calculate CAC by channel is:
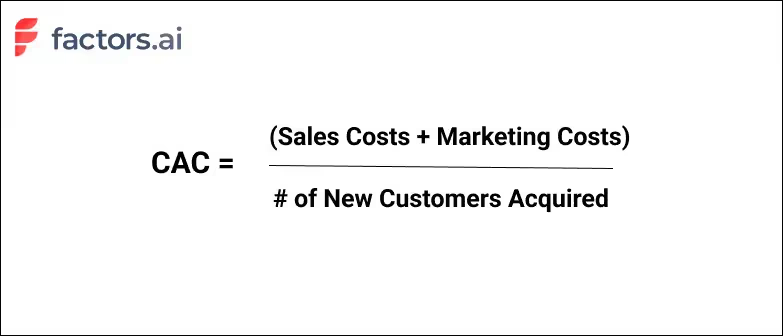
Why does this metric matter?
Measuring CAC lets you decide whether or not to pursue marketing for a particular channel and demonstrates high-performing marketing channels having low CAC.
4. Retention and churn rates
Customer retention and churn rates are opposites that indicate the number of customers retained or lost over a time period. The formula to calculate churn rate is:

Why are these metrics important?
Customer churn and retention rates are one of the most important metrics for SaaS CMOs. Measuring churn rate can help shed light on why subscribers are refusing to renew your service, and based on high or low retention rates, you can decide whether to pursue the same strategies or refresh them.
5. Customer lifetime value
Customer lifetime value, or CLV, indicates the average pay throughout a customer’s relationship with your company. It is a critical SaaS KPI to showcase revenue as it conveys a customer’s worth on average. The formula to calculate CLV is:
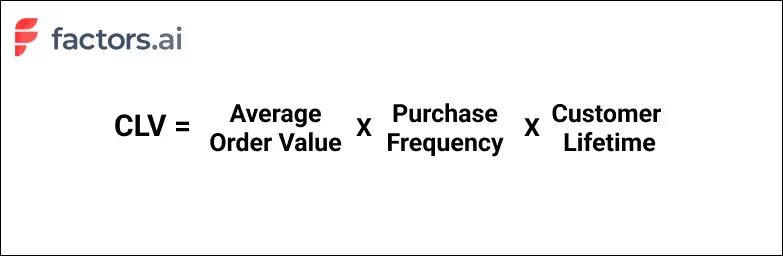
Why does this metric matter?
CLV is a critical SaaS business viability measure. It is essential for understanding the business value each customer brings and your SaaS company’s long-term potential.
6. Revenue and pipeline velocity by channel source
1. Revenue by channel source tracked by CMOs indicates how well their ads, campaigns, and other marketing efforts perform based on their source. Additionally, it tells you the aggregate revenue earned from successful conversions from each outlet.
2. Pipeline velocity by channel source is a key KPI indicating the speed at which leads move through your sales pipeline for each channel source.
Why do these metrics matter?
A high pipeline velocity means smoother onboarding processes and better conversions. So tracking this and revenue by channel source over various periods lets you improve conversions from each source.
7. Revenue Growth Percentage
Revenue growth percentage indicates how rapidly total company revenue increases over a set time, typically month-over-month or year-over-year. It shows the overall momentum and health of the business.
The formula to calculate revenue growth is straightforward—take the revenue difference between the current and past periods and divide that by the one-time period revenue.
For example, for year over year revenue growth, use the formula:
((Current Yearly Revenue - Last Year's Yearly Revenue) / Last Year's Yearly Revenue) x 100.
Why does this metric matter?
Monitoring rapid or declining growth % signals how well marketing and sales efforts are faring and hitting targets. When coupled with cost metrics, it also showcases profitability trends. Understanding growth by revenue streams also enables executives to double down on the highest traction channels.
8. Customer Lifetime Value
Customer lifetime value totals the likely earnings a business can generate from customers throughout their tenure. In essence, it conveys an average customer's worth.
The formula factors average order size, purchase frequency in a given period, and the average lifespan.
For instance, CLV may be calculated as: CLV = Average Order Value x Purchase Frequency Per Year x Average Lifespan in Years.
Why does this metric matter?
Tracking CLV over annual cohorts helps spotlight content, features, or channel innovations that make customers stickier. Comparing CLV vs acquisition cost also determines the breakeven period to recover expenses.
9. Cost Per Lead
Cost per lead signifies the average expenditure to generate a qualified marketing lead. It is calculated by dividing total lead gen marketing spend by the number of leads captured.
CPL can be calculated as: Total marketing dollars spent / Number of leads
If you spent $1000 to get 10 customers, that’s 1000/10 = $100 — Your CPL is $100.
Why does this metric matter?
Monitoring CPL trends determines the influence of brand equity on the sales funnel—a strong brand lowers CPL over time.
Comparing CPL by channel also helps optimize spending by identifying the lowest-cost customer acquisition sources. Analyzing CPL alongside customer lifetime value further ascertains profitable activities.
10. Brand Search Volume

Brand search volume measures monthly search queries for your brand name and keywords. Sudden surges or declines show changing consumer interest.
Why does this metric matter?
Tracks brand visibility gains/losses to correlate awareness-building campaigns and events. Guides keyword targeting and content strategies.
Creating Effective CMO Dashboards in Factors AI
Over the course of this article, we’ve covered what a CMO dashboard is, what its benefits are, and a few common metrics for CMOs to track. But constructing a comprehensive CMO dashboard is easier said than done — unless of course, you leverage a tailor-made dashboarding tool like Factors.
Factors unifies and reports data across complex buyer journeys involving multiple stakeholders, touchpoints, and channels under one roof. What does this mean for you? No more individual tools to analyze campaign performance, website engagement, and pipeline/revenue related metrics.
It’s simply all the metrics you care about as CMO in one, intuitive, customizable dashboard. Here are a few nifty features loved by CMOs:
- Ad hoc filters and breakdowns: Slice and dice metrics and KPIs with a wide range of filters and breakdowns to answer questions like: “How does conversion rate vary between companies with at least 200 employees and smaller teams of less than 50?” or “What’s the difference in sales velocity between leads from paid search and organic social?”
- Automated AI-fueled insights: It’s one thing to have your data in one place — but how can CMOs make sense of the numbers? Factors offers automated insights into what’s helping and hurting a specific conversion goal. What’s driving demo form submissions? Our Explain engine may suggest Linkedin campaigns and the pricing page have a positive influence while Display ads and email outreach is limiting performance. This helps the larger team improve resource allocation and drive ROI.
- Custom funnels, KPIs, and properties: Every business has different requirements. This is no different when it comes to reporting and dashboarding needs. Factors supports limitless customizations to KPIs and properties so CMO dashboards can be tailor-made to the what matters most to you.
Curious to see Factors in action? Schedule a personalized demo here!
.webp)
The Ultimate Guide to Marketing Analytics for SaaS Companies
Marketing analytics has become an integral part of any successful marketing strategy.
The industry is expected to grow at a CAGR of 14.8% between 2023 and 2028. Meanwhile, businesses of all sizes must spend the time to upgrade their in-house tech and employee skill-set to stay ahead of the curve.
But with the industry peppering buzzwords and jargon, marketing analytics can seem more complicated than it actually is.
In this guide, we’ll work to make the complex simpler. We’ll discuss what marketing analytics is, why it is necessary, which teams and people in a company should look into it, common limitations and pitfalls, and more. Let’s get started.
What is Marketing Analytics?
Marketing analytics refers to the analysis of data to gain insights into the performance of marketing campaigns and activities. It relies on the number of leads generated, conversion rates, customer acquisition cost (CAC), and customer lifetime value (LTV). Analyzing these metrics can help marketers and founders pave the way for marketing campaigns.
For instance, marketing analytics can help businesses understand which marketing channels or strategies are generating the most leads or conversions, and which ones may need improvement. It can also help identify trends and patterns in customer behavior, preferences, and buying habits, enabling businesses to make more informed decisions about their marketing efforts.
This is done through the use of tools like Google Analytics, Looker Studio, Factors.ai, and others for data visualization, statistical analysis, and predictive modeling.
The data is analyzed from various sources, such as website traffic, social media platforms, email campaigns, and Customer Relationship Management (CRM) systems.
The Benefits of Marketing Analytics
Marketing analytics offers multiple benefits in a B2B context. It helps businesses with interpreting data and making the most accurate and informed decisions. But that’s just one aspect of it. Let’s take a look at some other benefits of marketing analytics:
Improves Targeting
Marketing analytics enables you to identify the most relevant and receptive audience for your product or service. Then combining demographic, psychographic, and behavioral metrics, you can create a detailed customer persona that represents your ideal target audience. This data-driven approach allows you to tailor your messaging, offers, and content to resonate with these individuals, ultimately leading to higher conversion rates.

For instance, suppose your marketing analytics reveals that your most engaged customers are millennial entrepreneurs in the tech industry. In that case, you can fine-tune your marketing campaigns by focusing on the channels they frequent—such as LinkedIn and Twitter. Then begin creating content around industry trends, tips for startup growth, or case studies of successful tech entrepreneurs while subtly pitching your product or services.
Reduces Cost of Acquisition
The cost of acquiring a new customer can often exceed the expenses related to retaining an existing one. The average customer acquisition cost (CAC) including all SaaS industries is $704. Marketing analytics helps businesses identify the most cost-effective channels and methods for customer acquisition by studying metrics like cost-per-click, cost-per-lead, and return on ad spend.
.avif)
Let’s take an example here. Say your marketing analytics data shows that LinkedIn ads are generating a lower cost per acquisition (CPA) and higher conversion rate compared to Facebook ads. In this case, you can shift a larger portion of your ad budget to LinkedIn, driving down your overall cost of acquisition.
Improves the Customer Experience
86% of buyers are willing to pay more for brands that offer great customer experience. And SaaS companies doing $1 billion annually can expect to earn an additional $1 billion with just 3 years of investing in customer experience.
Analyzing customer feedback data can help SaaS marketers identify areas of improvement in their products or services. This leads to a better overall experience for customers. Marketing analytics can also help companies understand customer touchpoints, identify gaps in their communication strategies, and improve customer support.
Helps with Customer Retention
As time goes by, fewer and fewer customers stick around. This can be clearly demonstrated with the retention curve.
.avif)
However, spending money to retain existing customers is often more cost-effective than acquiring new ones.
Existing customers are 50% more likely to buy a product and spend 31% more on average than new customers. Marketing analytics can help businesses identify patterns and trends in customer behavior that may indicate potential churn or dissatisfaction.
For instance, if your marketing analytics reveals that customers who don't engage with your email campaigns are more likely to churn, you can modify your campaigns to launch a re-engagement strategy. You can target these at-risk customers with personalized content and offers to reignite their interest and loyalty. Sometimes, special pricing or preferential rates go a long way for customers.
Unveils Upselling Opportunities
Upselling is a powerful strategy in the B2B SaaS world. An average SaaS business generates 16% of its annual contract value (ACV) from upselling to customers. Companies doing $40 to $75 million annually can attribute twice as much revenue to upselling. With marketing analytics, you identify existing customers who are most likely to benefit from and be receptive to upgrading their current plans or purchasing additional products or services.
For instance, with marketing analytics data you may notice a few high-usage customers that could benefit from improved speed or better service offerings in your higher plans. Personalizing an upsell for those businesses and accounts can help you better improve your bottom lines in the long run.
What’s the Difference Between Product Analytics and Marketing Analytics?
Product analytics and marketing analytics are two important types of analytics that businesses need to use for making informed decisions. Unfortunately, these terms are used interchangeably and can cause confusion. Let’s look at the differences between product analytics and marketing analytics.

Product analytics analyzes the product experience. This type of analytics is generally performed on the data of users already using the product. It focuses on understanding how users interact with a product, including feature adoption, engagement, and product usage. With product analytics, the goal is to drive product-led growth by identifying areas for improvement, prioritizing feature development, and optimizing user experience.
Marketing analytics, on the other hand, helps identify how effective your marketing campaigns are. It helps identify how easily customers can find your product or website, what channels are working best for your marketing, and how many users are converting into paid users through the journey. Metrics for marketing analytics include lead generation, conversion rates, customer acquisition cost (CAC), and customer lifetime value (LTV).
For B2B businesses, both product and marketing analytics are important for driving growth. Product analytics helps businesses optimize product offerings and user experience. Marketing analytics helps companies improve their marketing strategies, target their audience more effectively, and allocate resources better.
Are Marketing Analytics and Marketing Attribution the Same Thing?
Marketing analytics and marketing attribution are complementary, yet distinct, disciplines within the realm of digital marketing. While both are essential for evaluating and optimizing marketing efforts, they serve different purposes and bring unique insights to the table. In this article, we will delve into the nuances of each concept, highlighting their primary focus, use cases, metrics, tools, and more.
Marketing Analytics vs Marketing Attribution - Quick Comparison
Marketing Analytics
Marketing analytics encompasses the measurement, management, and analysis of marketing performance data to optimize marketing effectiveness and maximize return on investment (ROI). Its primary focus is to provide a comprehensive understanding of marketing efforts, from the impact of individual campaigns to the overall health of a marketing strategy.
Use Cases:
- Identifying high-performing marketing channels
- Calculating customer acquisition cost (CAC) and customer lifetime value (LTV)
- Evaluating marketing ROI
- Tracking customer engagement and retention
- Analyzing data to inform marketing strategy and decision-making
Metrics:
Here are some of the marketing analytics metrics that you must keep a watch on.
- Customer acquisition cost (CAC): This metric helps you identify the cost of acquiring a new customer. It accounts for all the marketing and promotional costs involved in reaching and converting the customer.
- Lifetime value (LTV): Once a customer is converted, how long do they remain a paying customer? To find this value, note the length of time that each customer has stayed with your business and the total revenue generated by each. Then, multiply the average revenue per customer by the average length of time (lifespan). For example, if you have 100 customers paying, on average, $300 per month for 6 months, your customer lifetime value will be $300 x 6 = $1800.
- Churn Rate: How many of your customers are dropping off after they turn into paying customers? In fact, you can even count the churn rate for your free users as this can help you start digging deeper into the reasons why they don’t sign up for one of your paid plans.
- Retention Rate: This is the opposite of the churn rate. Here we check how many customers stick around for a specific period of time and more. For example, you may want to consider a customer as a retained one if they continue to pay for your SaaS for longer than 3 consecutive months.
- Return on investment: This metric is a must for businesses, no matter the industry. In the case of B2B SaaS businesses, this metric lets you understand how much money you are making based on the marketing spend.
Tools:
- Google Analytics: This is the most popular tool for marketing analytics—majorly because it’s free of cost. Google Analytics provides a wealth of information about traffic, user behavior, and much more.
- Factors: Factors is a powerful marketing analytics platform that specializes in B2B visitor identification, account analytics, and more. It integrates with your existing marketing stack and helps businesses reveal anonymous website visitors, decode customer journeys, and drive marketing ROI.
- Adobe Analytics: Adobe Analytics has a range of tools for tracking customer behavior, segmenting users, and identifying trends to improve overall marketing performance.
- Mixpanel: Mixpanel is more focused on product analytics. It enables businesses to monitor user interactions within web and mobile applications.
- Tableau: Tableau helps marketers transform raw marketing data into interactive visualizations that are easy to understand and share.
- HubSpot: HubSpot provides businesses with a holistic view of their performance and the tools necessary to optimize their strategies. From tracking website traffic and user behavior to measuring the effectiveness of email campaigns and social media outreach, it enables companies to make smarter marketing decisions and achieve better results.
Marketing Attribution
Marketing Attribution is the process of determining the value and impact of various touchpoints along the customer journey, helping marketers understand which channels, campaigns, and tactics are most effective in driving conversions and other desired outcomes. Attribution primarily focuses on assigning credit to specific marketing efforts that contribute to a conversion, sale, or other predefined goals.
Use Cases:
- Evaluating the effectiveness of individual marketing channels and campaigns
- Identifying the most influential touchpoints in the customer journey
- Optimizing marketing spend and resource allocation
- Informing marketing strategy and tactics based on attribution insights
- Tailoring messaging and targeting to maximize conversion potential
Attribution Models:
There are several attribution models available that help businesses assess the impact of their marketing efforts. Here is a breakdown of some of the most common types:
- Single-Touch Attribution: Assigns credit to a single touchpoint, either the first touch or the last touch. Common single-touch models include First-Touch Attribution, Last-Touch Attribution, and Last Non-Direct Touch Attribution.
- Multi-Touch Attribution: Distributes credit across multiple touchpoints throughout the customer journey. Examples of multi-touch models are Linear Attribution, U-Shaped Attribution, and Time Decay Attribution.
- Position-Based Attribution: This attribution model allocates credits based on the position of a touchpoint in the customer journey. For instance, it gives 40% of the credit to both the first and last ad interactions and corresponding keywords. The remaining 20% is spread across the other ad interactions on the user journey.
- Custom Attribution: Utilizes data and machine learning algorithms to create custom attribution models tailored to the unique needs of a specific business or industry. This approach allows for a more accurate representation of the customer journey and the impact of various marketing channels and tactics.
Tools:
- Factors: Factors is an AI-driven marketing attribution platform that provides detailed insights into the impact of each touchpoint on your customer journey. With its advanced modeling capabilities, Factors helps businesses make informed decisions on marketing spend and resource allocation.
- Adobe Marketo Measure: A part of the Adobe Marketo suite, Measure offers powerful marketing attribution and revenue planning features. By connecting marketing and sales data, Measure enables businesses to accurately attribute revenue to specific marketing efforts.
- Dreamdata: Dreamdata is a B2B revenue attribution platform that allows you to track and analyze the performance of your marketing efforts across all channels. By unifying data from various sources, Dreamdata provides a holistic view of your customer journey and helps optimize your marketing strategy.
- Ruler Analytics: Ruler Analytics is a marketing attribution platform that connects closed revenue data with the customer journey. It provides insights into which marketing channels are driving conversions, allowing businesses to optimize their efforts and improve ROI.
Who Should Be Thinking About Marketing Analytics at SaaS Companies?
Marketing analytics helps businesses measure the effectiveness of their marketing campaigns, optimize strategies, and make data-backed decisions to drive growth. But who should be thinking about marketing analytics at SaaS companies? Generally, it is CMOs, marketing executives, and CROs who stay updated with this information.

But, there's more to the story.
While it's essential for top-level executives and decision-makers to understand marketing metrics, it's not practical for everyone involved to monitor every single metric. Requiring the marketing team to constantly share their progress is counter-intuitive to progress as well. That’s where dashboards help teams stay in sync and keep tabs on what’s happening within marketing.
Dashboards offer a streamlined and efficient way to stay on top of the effectiveness of marketing strategies. They present data in a visually appealing and easy-to-understand format, allowing for quick insights and adjustments when needed. Here are a few benefits of dashboards.
- Enhanced collaboration: A well-designed dashboard helps teams across the board to stay updated with the changes in marketing. It also reduces the communication required to get data from individual teams as everyone can directly pull data from the dashboards. This increases transparency between teams.
- Increased accountability: Because of the increased transparency, teams, and individuals have accountability for the tasks assigned to them. This allows for greater productivity across teams and helps things progress faster.
- Customization: Dashboards are designed to be completely customizable. You do not need to showcase the same verbose data to everyone involved. Dashboards can help you summarize and visualize the required data based on who you want to demonstrate or showcase the data to.
If you want to implement a marketing dashboard within your company, we have an in-depth article on CMO dashboards. This will guide you through the process of designing a dashboard that serves the unique needs of your SaaS app development company.
Addressing Common Marketing Analytics Challenges
As businesses continue to evolve and adapt to the digital era, marketing analytics has become a vital aspect of marketing success. However, there are several common challenges that marketers face when trying to harness the power of data analysis.
Siloed data
The importance of seamless data integration cannot be overstated. With various marketing tools and platforms being used simultaneously, data can become fragmented and difficult to consolidate. Apart from that, customer data can get siloed at each of the touchpoints as well.
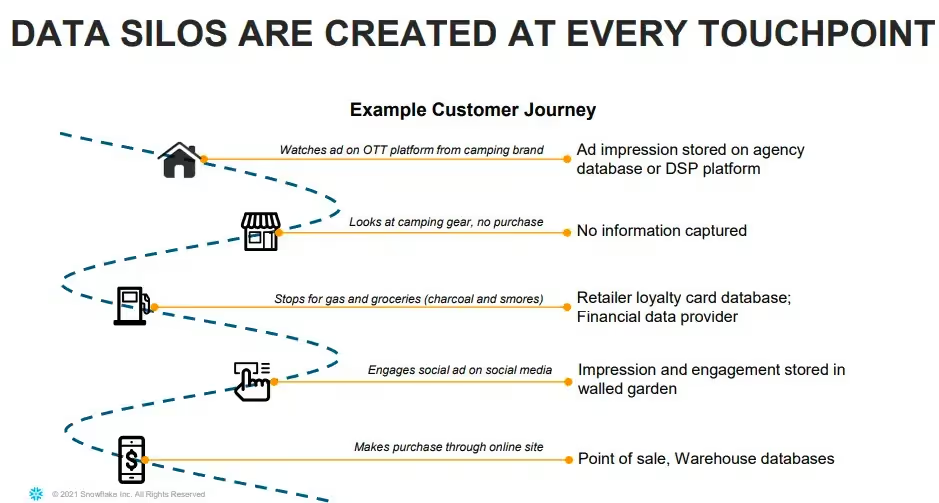
This siloed data can lead to an incomplete view of your marketing efforts and hinder your ability to make data-driven decisions. Marketing attribution tools like Factors combine data from multiple platforms and create a unified timeline that helps you view all the touchpoints of your customers in a single place.
Not knowing what to track
Identifying the right metrics to track is essential for marketing success. Focusing on the wrong metrics or not tracking them at all can lead to misguided decision-making. In fact, tracking a metric incorrectly can lead to worse outcomes than not tracking it at all.
For example, if you incorrectly attribute the success of your conversions to your paid ads, you may end up spending more on the wrong channel and wasting your marketing efforts.
Incorrect understanding of metrics
Metrics should be analyzed in tandem with other data to gain meaningful insights. For example, if the cost of acquisition in one quarter is dramatically lower than that of the previous two quarters, it could be the direct result of pausing a poor-performing marketing campaign.
But only looking at the CAC may lead to incorrect conclusions—CAC is going lower and thus your profits are going up.
Lack of automation and limited visualizations
Manual data analysis can be time-consuming, and error-prone, and ultimately limit your ability to make the most of it. Automating data collection and analysis processes is essential for maximizing efficiency and accuracy. Also, visualizing data through intuitive dashboards and reports enables easier understanding for everyone involved.
Factors address these common challenges by offering a powerful marketing analytics platform featuring seamless integrations, automation, and advanced visualization capabilities. Comprehensive marketing analytics and attribution solutions like Factors help overcome these challenges and ultimately achieve better results from your marketing efforts.
Top Marketing Analytics Use Cases for SaaS Companies
Marketing analytics can provide valuable insights and drive growth in various aspects of a SaaS company’s marketing efforts. The three key use cases for marketing analytics include acquisition and conversion tracking, campaign performance analysis, and customer segmentation and personalization. Here’s what each of them means:
1. Acquisition and Conversion Tracking
Acquisition and conversion tracking allows SaaS businesses to monitor how efficiently their marketing efforts are attracting and converting customers. For example, a SaaS company offering project management software might use acquisition and conversion tracking to analyze the sources of their website traffic. This could include social media platforms, search engines, and email marketing campaigns. They can then determine which channels are most effective in driving new users to sign up for their software. If the analysis reveals that content and SEO are generating a high number of sign-ups, the company can allocate more resources to that platform to capitalize on its success.
2. Campaign Performance Analysis
Campaign performance analysis evaluates the effectiveness of marketing campaigns and helps improve the campaigns through data. This involves monitoring click-through rates, conversion rates, and cost per acquisition, to determine the overall success of each campaign.
Imagine a SaaS company specializing in human resources software launches a Google Ads campaign targeting HR managers.
Campaign performance analysis can be used here to evaluate the effectiveness of the ads. If the data indicates that one type of ad is outperforming the others, the company can optimize the campaign by allocating a higher budget to the more successful ad. This constant analysis and improvement can lead to more efficient marketing campaigns and higher ROI.
3. Customer Segmentation and Personalization
Customer segmentation and personalization involve grouping customers based on shared characteristics and tailoring marketing messages and offers to meet their specific needs.
For instance, a SaaS company that provides email marketing software could segment its customers into groups based on their industry, company size, or the frequency of their email campaigns.
They could then create content and offers targeted at each segment. A small e-commerce business might receive tips on how to maximize its email open rates during the holiday season. A large enterprise client, on the other hand, might receive a case study showcasing how their email marketing success has successfully scaled email campaigns for similar organizations.
Grow your business with streamlined marketing analytics
Marketing analytics is a powerful tool for driving growth and improving marketing performance in B2B companies. Focusing on key use cases, such as acquisition and conversion tracking, campaign performance analysis, and customer segmentation and personalization, will enable you to make data-driven decisions and optimize your marketing efforts.
As a result, you'll attract more customers, improve customer satisfaction, and ultimately, increase your company's revenue. Don't miss out on the potential that marketing analytics can unlock for your SaaS company—start harnessing the power of data with Factors.ai today.
FAQs
Why is marketing analytics important?
Marketing analytics makes marketing effective. It offers deep insights into the preferences and behavior of your customers. It allows you to shape the marketing campaigns in accordance with your customer’s expectations and helps you make data-backed decisions rather than relying on intuition and experience.
What are the three types of analytics that can be used in marketing?
Descriptive analytics, predictive analytics, and prescriptive analytics.
- Descriptive analytics analyzes historical data to understand past performance.
- Predictive analytics uses data and statistical algorithms to forecast future outcomes
- Prescriptive analytics provides recommendations on how to optimize marketing efforts based on data-driven insights.

Identify & Target High-Intent Accounts With Webhooks & Pipedrive
Target the right accounts, at the right time with intent-based outreach
B2B sales teams spend a lot of time and effort reaching out to cold prospects only to achieve disappointing results. In fact, even successful benchmarks tag the average cold-call response rate at just 2%.
And honestly, It’s not difficult to see why.
While it’s simple enough to find lists of companies and contacts that fit your ideal client profile, it’s a monumental challenge to convince prospects to consider your solution when they’re not in the market for one.
So what’s the alternative to reaching out to the right accounts at the wrong time?
Reaching out to the right accounts at the right time of course! Or more specifically, it’s intent-based outreach based on the goldmine of anonymous, sales-ready companies already visiting your website.
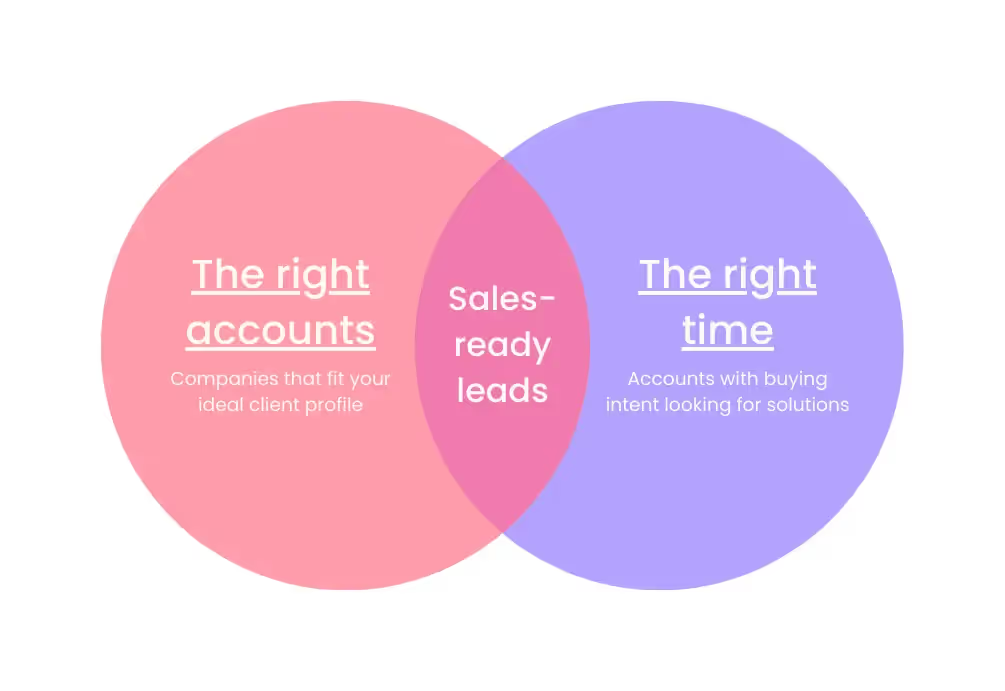
The following guide explores how to identify and target sales-ready accounts with the combined powers of Factors’ account identification and Pipedrive webhooks. We first discuss how this integration works, before delving into a handful of use-cases.
How It Works: Pushing data back into Pipedrive
Factors taps into industry-leading IP-lookup technology to identify up to 64% of anonymous website traffic at an account-level — without the need for form submissions. This includes company names as well as firmographics such as geography, industry, employee headcount, revenue range and more.

In addition, Factors auto-tracks account-level website activity, engagement, and intent with advanced analytics. This includes page views, button clicks, scroll-depth, account timelines, funnels and more.
With this information, users can filter the total set of anonymous website visitors down to ICP accounts that have expressed buying intent:
- ICP criteria: Filter down traffic based on firmographics such as industry, headcount and revenue-range to identify accounts that fit your ideal client profile.
- Intent criteria: Filter down traffic based on intent signals such as high-intent page views such as pricing, time-spent on page, and percentage scroll-depth to identify sales-ready buyers.
In short, access a list of high-intent ICP accounts that are already visiting your website but are yet to submit a form or sign-up.
Now, with webhooks and Zapier, it’s easier than ever to automatically push all this identification data from Factors into any other tool your team uses. This includes ad platforms, marketing automation platforms, and, in this case, Pipedrive CRM.
How will this help? Rather than going after cold leads with negligible chances of conversion, sales reps can view, segment, and target sales-ready accounts inside Pipedrive. As we’ll see in the next section, this dramatically simplifies and improves targeted sales outreach.

Implementing Webhooks on Factors is easy as pie. See how here.
Use-cases: Making the most of your website traffic
1. Identify new business opportunities
Factors surfaces anonymous, high-intent companies visiting your website. As previously discussed, this data can be filtered down to high-fit, high-intent accounts.
Using webhooks, this data can be pushed from Factors into Pipedrive. In other words, you can automatically create organizations inside Pipedrive for visitors that match your ICP and intent criteria.
For example, webhooks can be configured to create a new company when a visitor from a US-based software company with at least 250 employees is live on your website.
Here are a few more examples of what you can see inside your CRM with Factors:
- Accounts that visit a landing page through a search ad but fail to submit a form
- Software companies with at least 500 employees visiting high-intent pages like pricing
- US-based companies that have read through at least half a product comparison blog
Rather than relying on the 5% of website traffic that submits a form, teams can identify and target a deep new pool of potential pipeline — all within Pipedrive. What’s more? Alerts can be relayed to sales reps in real-time through Slack or MS teams so they can immediately reach out to live prospects.

2. Stay on top of existing target accounts
In addition to recording new accounts visiting your website, Factors can be used to monitor and update data for target accounts that already exist within Pipedrive.
For example, say an account clicks on a search ad, submits a demo form, but never schedules time on your calendar. While the account's data is available in Pipedrive, it can be tedious to track and update their actions post the demo form submission.
To solve for this, Factors can automatically update CRM properties based on trigger criterias when account return to your website. Let’s say that the same account is back reading a product alternatives blog or visiting the pricing page after a couple of weeks. This event can be updated within Pipedrive, including their last active time.

Sales reps can be notified with real-time when high-intent events take place so as to be able to immediately reach out to accounts and improve the odds of conversion.
3. Accelerate deals with behavioral data
Certain marketing material may or may not be relevant depending on the audience in question. For example, an enterprise-level account may be especially interested in security compliance related content. An early-stage start-up, on the other hand, may find content around cost-effective pricing more appealing.
Factors can track how various types of companies are interacting with your website to understand what target accounts care about most. This data can be pushed back into Pipedrive so sales reps can easily assess a prospect’s interactions, priorities and pain-points before jumping into a sales call.
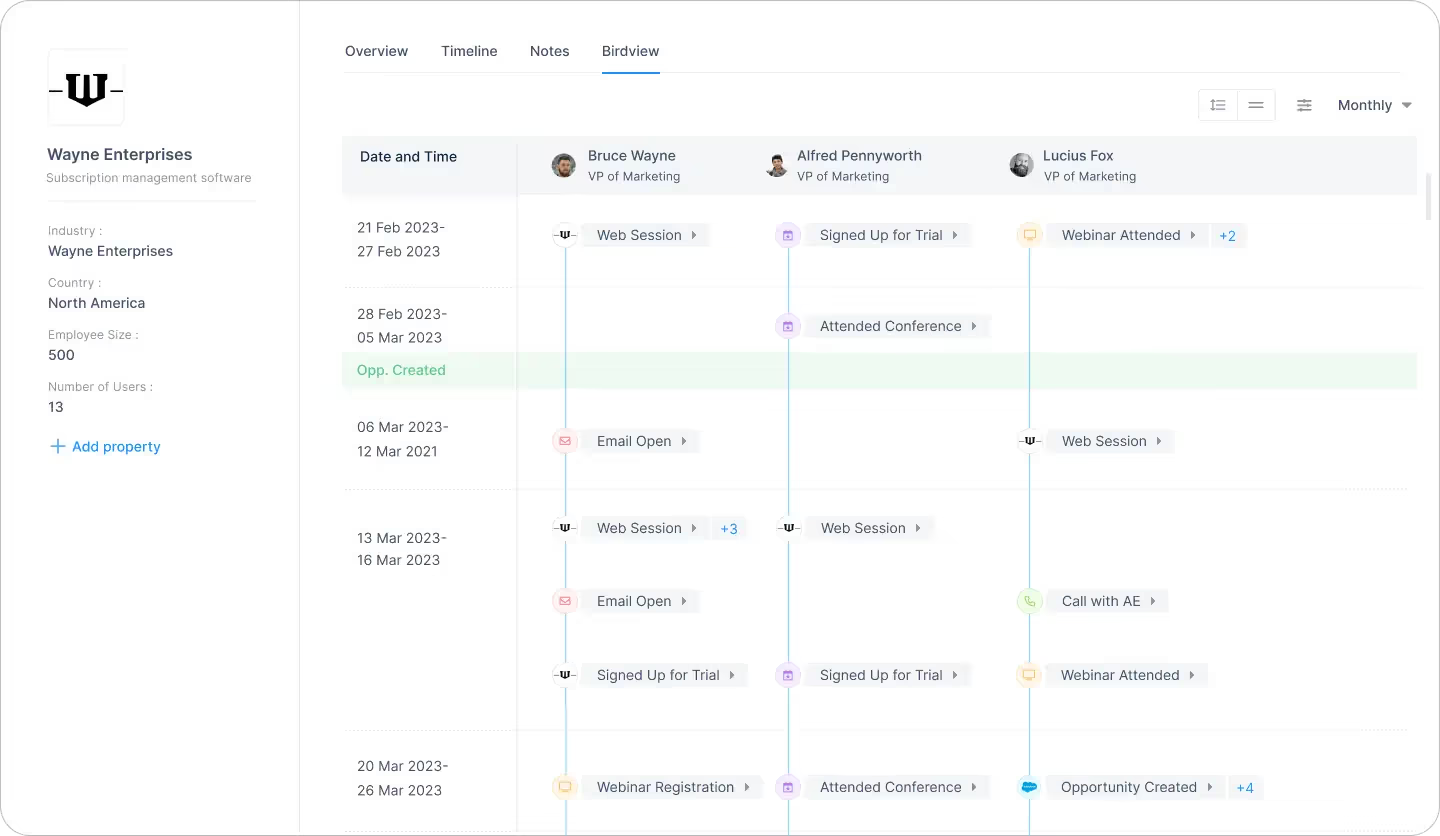
For one, sales reps can accelerate deals by personalizing the customer experience. For another, marketing teams can gauge what resonates best with the target audience and finetune content efforts accordingly.
4. Rekindle lost opportunities
Use Factors to track how prospects who have dropped off the funnel or former customers are returning to engage with your website. For instance, maybe a client who churned a couple of quarters ago is back interacting with a page that highlights a new feature release.
This may be an intent-signal that the account is reconsidering your product. It might be a good idea for sales reps to reach out and share some relevant information on what’s new. Of course, this doesn’t necessarily guarantee a conversion. But it’s far more effective than reaching out to an ice cold prospect.
This guide has covered a handful of ways in which pushing visitor data back into Pipedrive can be helpful. Ultimately, the goal is to align account data with relevant stakeholders and technologies in order to:
- Drive intent-based sales outreach
- Refine ABM efforts and spends
- Optimize retargeting campaigns
There are countless other use-cases with account identification working in conjunction with CRMs, MAPs, and more. With webhooks, Factors can push valuable website account data to nearly any platform on the planet. How you make the most of that data is really up to you — the possibilities are endless.
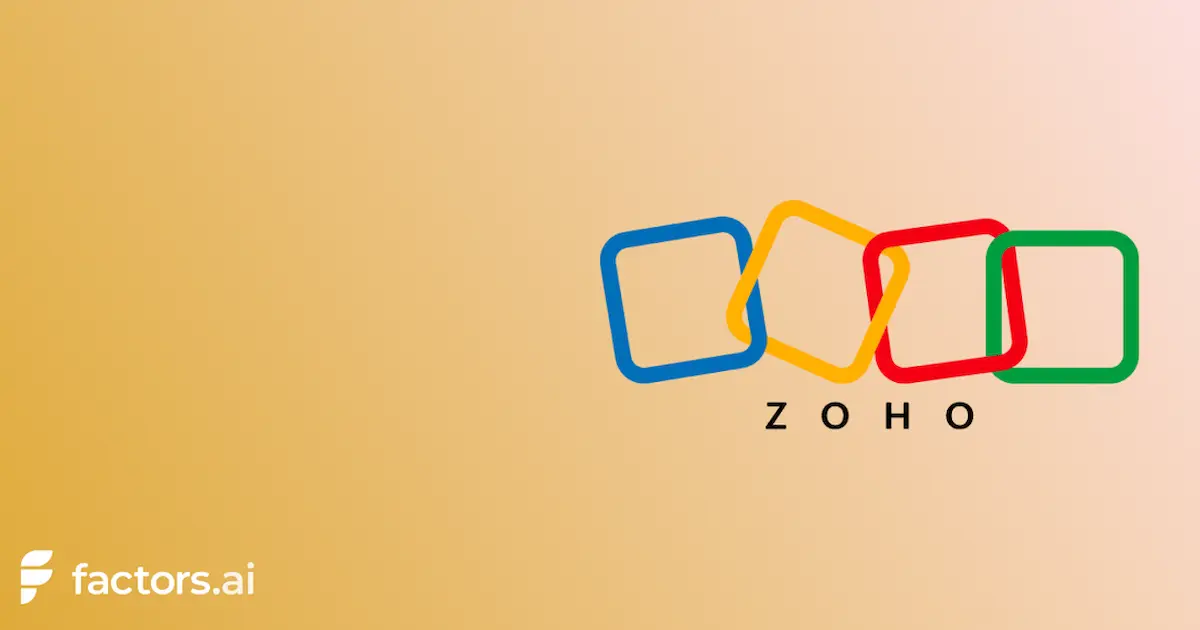
Discover Sales-Ready Accounts With Zoho & Webhooks
Target the right accounts, at the right time with intent-based outreach
B2B sales teams spend a lot of time and effort reaching out to cold prospects only to achieve disappointing results. In fact, even successful benchmarks tag the average cold-call response rate at just 2%.
And honestly, It’s not difficult to see why.
While it’s simple enough to find lists of companies and contacts that fit your ideal client profile, it’s a monumental challenge to convince companies to consider your solution when they’re not in the market for one.
So what’s the alternative to reaching out to the right accounts at the wrong time?
Reaching out to the right accounts at the right time of course! Or more specifically, it’s intent-based outreach based on the goldmine of anonymous, sales-ready companies already visiting your website.

The following guide explores how to identify and target sales-ready accounts with the combined powers of Factors’ account identification and Zoho webhooks. We first discuss how this integration works, before delving into a handful of use-cases.
How It Works: Pushing visitor data back into Zoho
Factors taps into industry-leading IP-lookup technology to identify up to 64% of anonymous account visiting your website. This includes company names as well as firmographics such as geography, industry, employee headcount, revenue range and more.
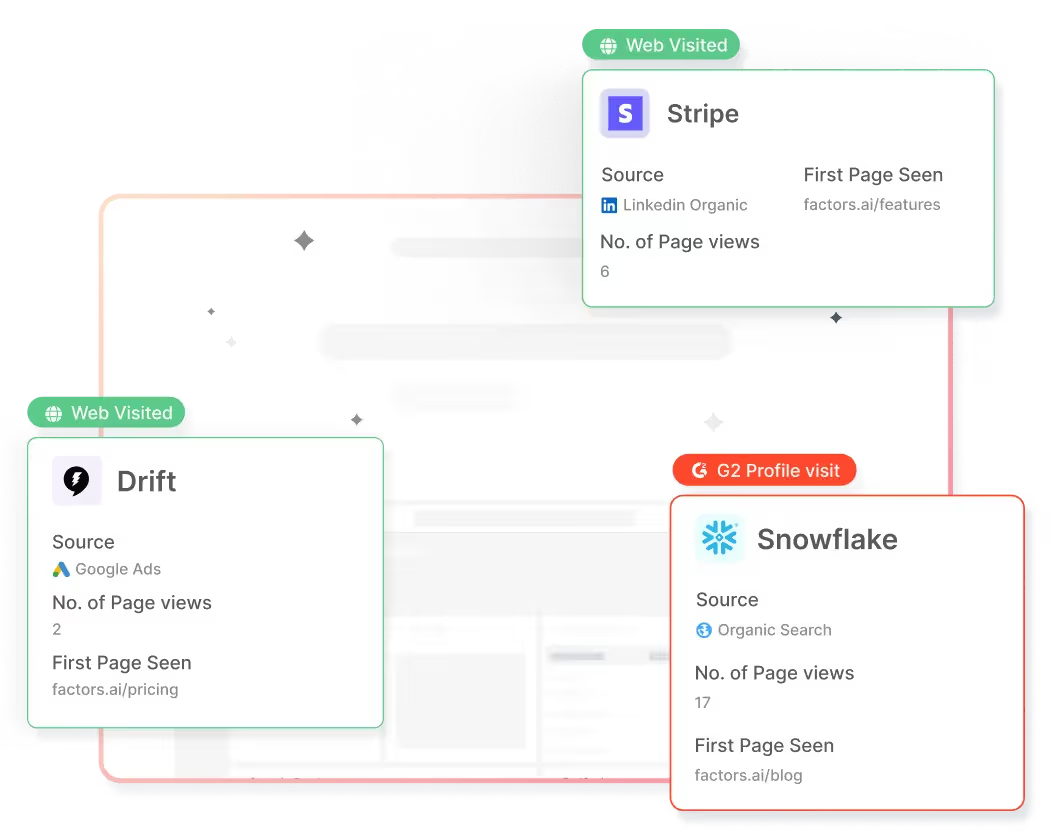
In addition, Factors auto-tracks website activity and engagement at an account level with advanced analytics. This includes page views, button clicks, scroll-depth, account timelines, funnels and more.
With this information, users can filter the total set of anonymous traffic down to ICP accounts that have expressed buying intent:
- ICP criteria: Filter down traffic based on firmographics such as industry, headcount and revenue-range to identify accounts that fit your ideal client profile.
- Intent criteria: Filter down traffic based on intent signals such as high-intent page views such as pricing, time-spent on page, and percentage scroll-depth to identify sales-ready buyers.
In short, access a list of high-intent ICP accounts that are already visiting your website but are yet to submit a form or sign-up.
Now, with webhooks and Zapier, it’s easier than ever to automatically push all this data from Factors into any other tool your team uses. This includes ad platforms, marketing automation platforms, and, in this case, Zoho CRM.
How will this help? Rather than going after cold leads with negligible chances of conversion, sales reps can view, segment, and target sales-ready visitors inside Zoho. As we’ll see in the next section, this dramatically simplifies and improves targeted sales outreach.
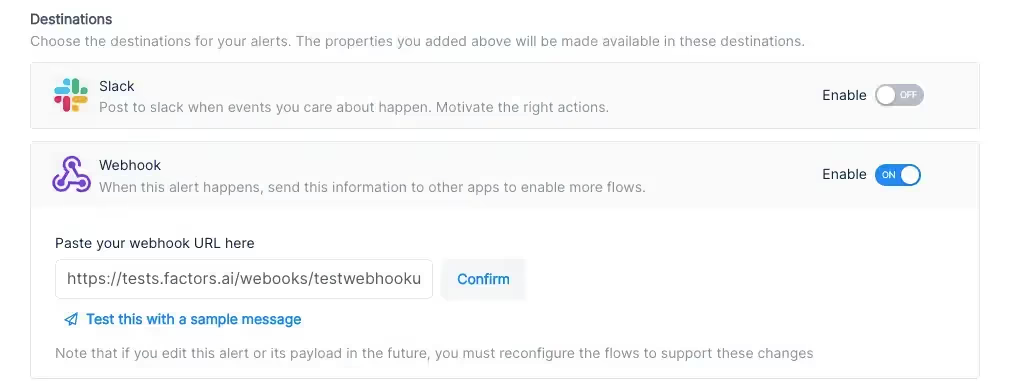
Implementing Webhooks on Factors is easy as pie. See how here.
Use-cases: Making the most of your website visitors
1. Identify new business opportunities
Factors surfaces anonymous, high-intent companies visiting your website — even if they’re yet to submit a contact form. As previously discussed, this data can be filtered down to high-fit, high-intent accounts.
Using webhooks, this data can be pushed from Factors into Zoho. In other words, you can automatically create accounts inside Zoho for companies that match your ICP and intent criteria.
For example, webhooks can be configured to create a new company when a visitor from a US-based software company with at least 250 employees is live on your website.
Here are a few more examples of what you can see inside your CRM with Factors:
- Accounts that visit a landing page through a search ad but fail to submit a form
- Software companies with at least 500 employees visiting high-intent pages like pricing
- US-based companies that have read through at least half a product comparison blog
Rather than relying on the 5% of website traffic that submits a form, teams can identify and target a deep new pool of potential pipeline — all within Zoho. What’s more? Alerts can be relayed to sales reps in real-time through Slack or MS teams so they can immediately reach out to live prospects.

2. Stay on top of existing target accounts
In addition to recording new accounts visiting your website, Factors can be used to monitor and update data for target accounts that already exist within Zoho.
For example, say an account ad clicks on a search ad, submits a demo form, but never schedules time on your calendar. While the account's data is available in Zoho, it can be tedious to track and update their actions post the demo form submission.
To solve for this, Factors can automatically update CRM properties based on trigger criterias when accounts return to your website. Let’s say that the same account is back reading a product alternatives blog or visiting the pricing page after a couple of weeks. This event can be updated within Zoho, including their last active time.
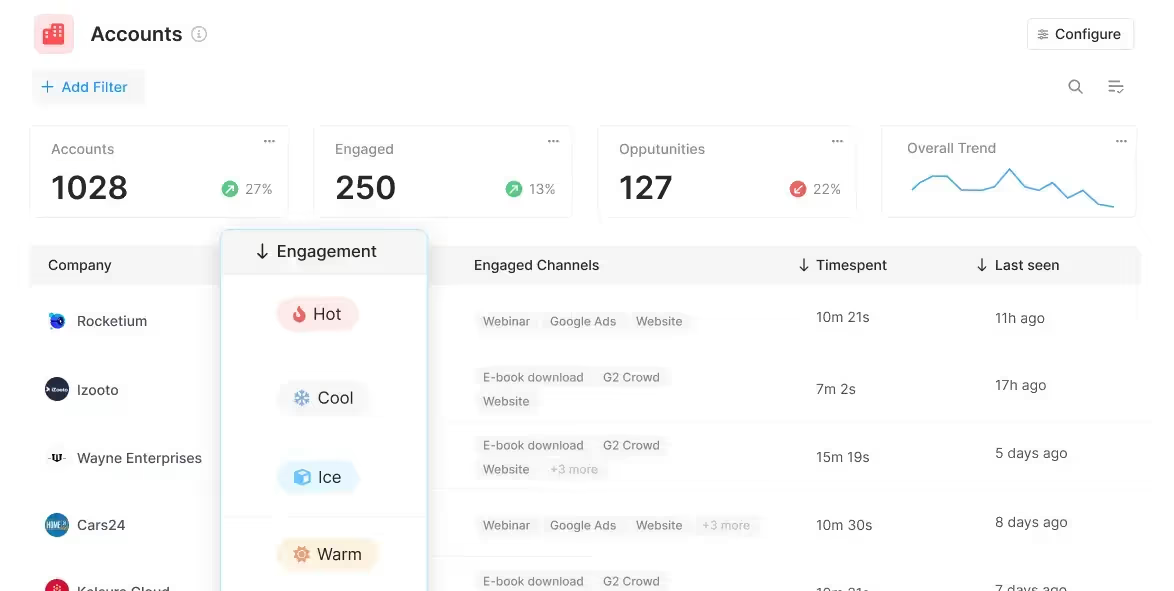
Sales reps can be notified with real-time when high-intent events take place so as to be able to immediately reach out to target accounts and improve the odds of conversion.
3. Accelerate deals with behavioral data
Certain marketing material may or may not be relevant depending on the audience in question. For example, an enterprise-level account may be especially interested in security compliance related content. An early-stage start-up, on the other hand, may find content around cost-effective pricing more appealing.
Factors can track how various types of companies are interacting with your website to understand what target accountscare about most. This data can be pushed back into Zoho so sales reps can easily assess a prospect’s interactions, priorities and pain-points before jumping into a sales call.

For one, sales reps can accelerate deals by personalizing the customer experience. For another, marketing teams can gauge what resonates best with the target audience and finetune content efforts accordingly.
4. Rekindle lost opportunities
Use Factors to track how accounts that have dropped off the funnel or former customers are returning to engage with your website. For instance, maybe a client who churned a couple of quarters ago is back interacting with a page that highlights a new feature release.
This may be an intent-signal that the account is reconsidering your product. It might be a good idea for sales reps to reach out and share some relevant information on what’s new. Of course, this doesn’t necessarily guarantee a conversion. But it’s far more effective than reaching out to an ice cold prospect.
This guide has covered a handful of ways in which pushing account data back into Zoho can be helpful. Ultimately, the goal is to align account data with relevant stakeholders and technologies in order to:
- Drive intent-based sales outreach
- Refine ABM efforts and spends
- Optimize retargeting campaigns
There are countless other use-cases with account identification working in conjunction with CRMs, MAPs, and more. With webhooks, Factors can push valuable account data to nearly any platform on the planet. How you make the most of that data is really up to you — the possibilities are endless.

Convert High-Intent Accounts With Salesforce & Webhooks
Target the right accounts, at the right time with intent-based outreach
B2B sales teams invest significant time and resources into reaching out to prospects who are yet to show any intention of buying. However, this cold outreach almost always yields disappointing results. Even the most comprehensive benchmarks indicate that the average response to cold-calls is only 2%.
And honestly, It’s not difficult to see why.
While it’s easy enough to find lists of companies and leads that fit your ideal client profile, it’s extremely challenging to convince prospects to consider your solution when they’re not yet ready to buy.
So what’s the alternative to reaching out to the right accounts at the wrong time?
Reaching out to the right accounts at the right time of course! Or more specifically, it’s intent-based outreach based on the pot of gold that is the anonymous, sales-ready companies already visiting your website.
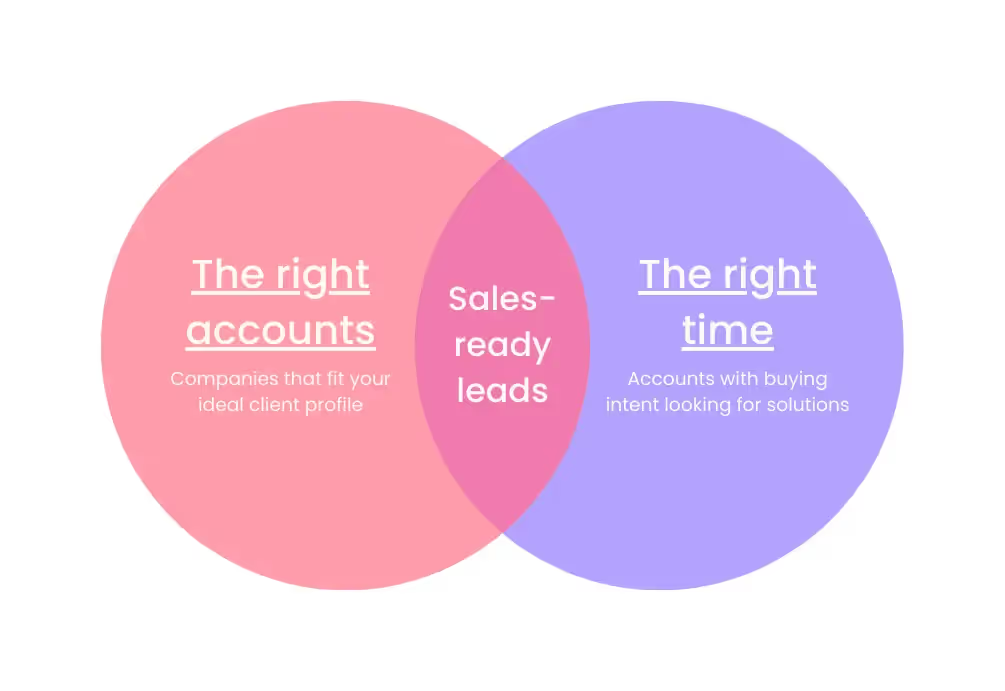
The following guide explores how to identify and convert high-intent accounts with the combined powers of Factors’ accounts identification and Salesforce webhooks. We first discuss how this integration works, before delving into a handful of use-cases.
How It Works: Pushing website data back into Salesforce
Factors taps into industry-leading IP-lookup technology to identify up to 64% of anonymous companies visiting your website — without the need for form submissions. This includes company names as well as firmographics such as geography, industry, employee headcount, revenue range and more.
In addition, Factors auto-tracks website activity and engagement at an accounts level with advanced analytics. This includes page views, button clicks, scroll-depth, account timelines, funnels and more.

With this information, users can filter the total set of anonymous traffic down to ICP accounts that have expressed buying intent:
- ICP criteria: Filter down traffic based on firmographics such as industry, headcount and revenue-range to identify accounts that fit your ideal client profile.
- Intent criteria: Filter down traffic based on intent signals such as high-intent page views such as pricing, time-spent on page, and percentage scroll-depth to identify sales-ready buyers.
In short, access a list of high-intent ICP accounts that are already visiting your website but are yet to submit a form or sign-up.
Now, with webhooks and Zapier, it’s easier than ever to automatically push all this account data from Factors into any other tool your team uses. This includes ad platforms, marketing automation platforms, and, in this case, Salesforce CRM.
How will this help? Rather than going after cold leads with negligible chances of conversion, sales reps can view, segment, and target sales-ready accounts inside Salesforce. As we’ll see in the next section, this dramatically simplifies and improves targeted sales outreach.

Implementing Webhooks on Factors is easy as pie. See how here.
Use-cases: Making the most of your website traffic
1. Identify new business opportunities
Factors surfaces anonymous, high-intent companies visiting your website. As previously discussed, this data can be filtered down to high-fit, high-intent accounts.
Using webhooks, this data can be pushed from Factors into Salesforce. In other words, you can automatically create accounts inside Salesforce for companies that match your ICP and intent criteria.
For example, webhooks can be configured to create a new account when a visitor from a US-based software company with at least 250 employees is live on your website.
Here are a few more examples of what you can see inside your CRM with Factors:
- Accounts that visit a landing page through a search ad but fail to submit a form
- Software companies with at least 500 employees visiting high-intent pages like pricing
- US-based companies that have read through at least half a product comparison blog
Rather than relying on the 5% of website traffic that submits a form, teams can identify and target a deep new pool of potential pipeline — all within Salesforce. What’s more? Alerts can be relayed to sales reps in real-time through Slack or MS teams so they can immediately reach out to live prospects.

2. Stay on top of existing target accounts
In addition to recording new accounts visiting your website, Factors can be used to monitor and update data for target accounts that already exist within Salesforce.
For example, say an account clicks on a search ad, submits a demo form, but never schedules time on your calendar. While the account's data is available in Salesforce, it can be tedious to track and update their actions post the demo form submission.
To solve for this, Factors can automatically update CRM properties based on trigger criteria when leads return to your website. Let’s say that the same account is back reading a product alternatives blog or visiting the pricing page after a couple of weeks. This event can be updated within Salesforce, including their last active time.

Sales reps can be notified with real-time when high-intent events take place so as to be able to immediately reach out to leads and improve the odds of conversion.
3. Accelerate deals with behavioral data
Certain marketing material may or may not be relevant depending on the audience in question. For example, an enterprise-level account may be especially interested in security compliance related content. An early-stage start-up, on the other hand, may find content around cost-effective pricing more appealing.
Factors can track how various types of companies are interacting with your website to understand what visitors care about most. This data can be pushed back into Salesforce so sales reps can easily assess a prospect’s interactions, priorities and pain-points before jumping into a sales call.
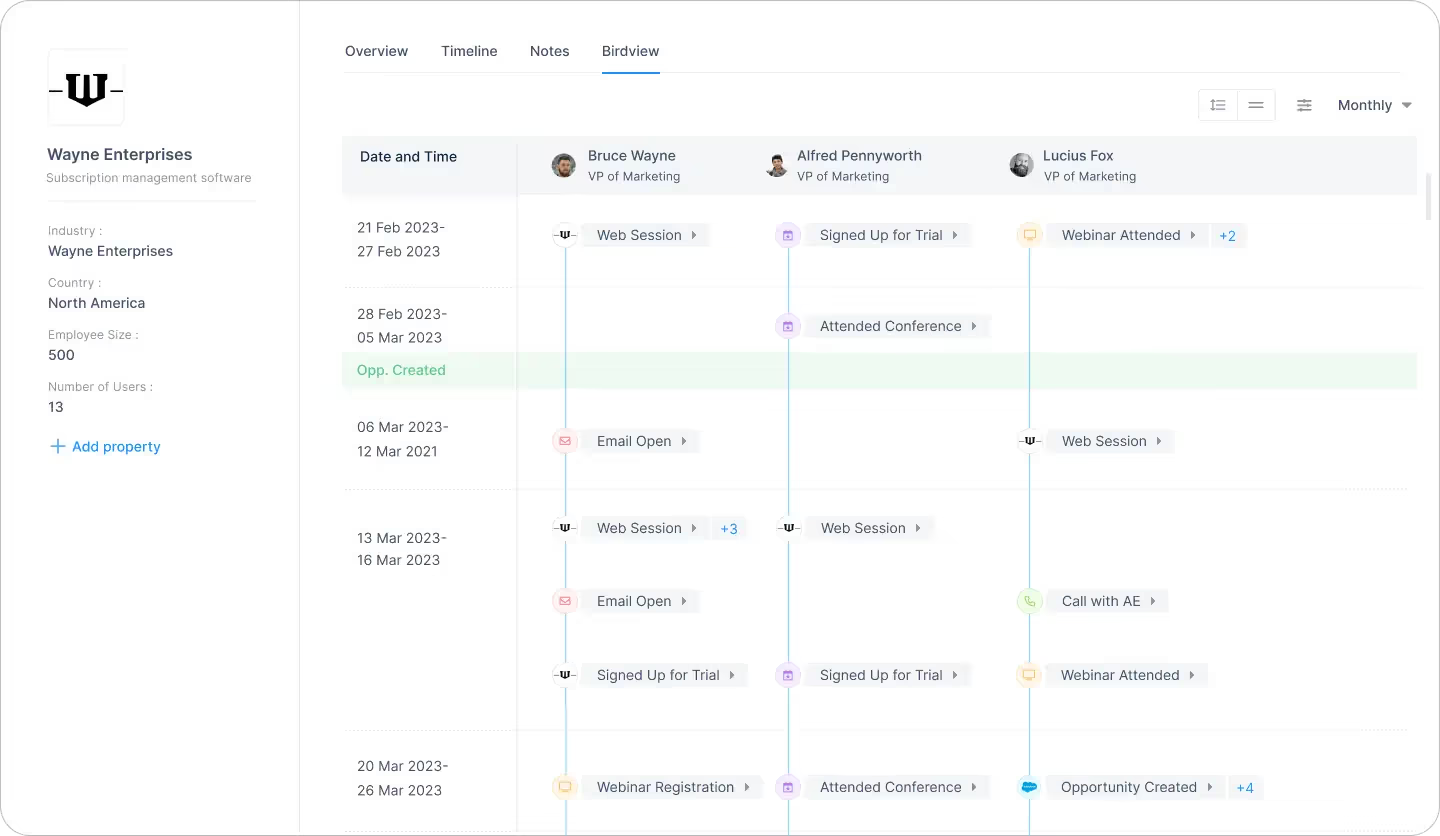
For one, sales reps can accelerate deals by personalizing the customer experience. For another, marketing teams can gauge what resonates best with the target audience and fine-tune content efforts accordingly.
4. Rekindle lost opportunities
Use Factors to track how accounts that have dropped off the funnel or former customers are returning to engage with your website. For instance, maybe an account that churned a couple of quarters ago is back interacting with a page that highlights a new feature release.
This may be an intent-signal that the lead is reconsidering your product. It might be a good idea for sales reps to reach out and share some relevant information on what’s new. Of course, this doesn’t necessarily guarantee a conversion. But it’s far more effective than reaching out to an ice cold lead.
This guide has covered a handful of ways in which pushing account data back into Salesforce can be helpful. Ultimately, the goal is to align visitor data with relevant stakeholders and technologies in order to:
- Drive intent-based sales outreach
- Refine ABM efforts and spends
- Optimize retargeting campaigns
There are countless other use-cases with account identification working in conjunction with CRMs, MAPs, and more. With webhooks, Factors can push valuable account data to nearly any platform on the planet. How you make the most of that data is really up to you — the possibilities are endless.

Identify Sales-Ready Account With Hubspot & Webhooks
Target the right accounts, at the right time with intent-based outreach
B2B sales teams spend a lot of time and effort reaching out to cold prospects only to achieve disappointing results. In fact, even successful benchmarks tag the average cold-call response rate at just 2%.
And honestly, It’s not difficult to see why.
While it’s simple enough to find lists of companies and contacts that fit your ideal client profile, it’s a monumental challenge to convince prospects to consider your solution when they’re not in the market for one.
So what’s the alternative to reaching out to the right accounts at the wrong time?
Reaching out to the right accounts at the right time of course! Or more specifically, it’s intent-based outreach based on the goldmine of anonymous, sales-ready companies already visiting your website.
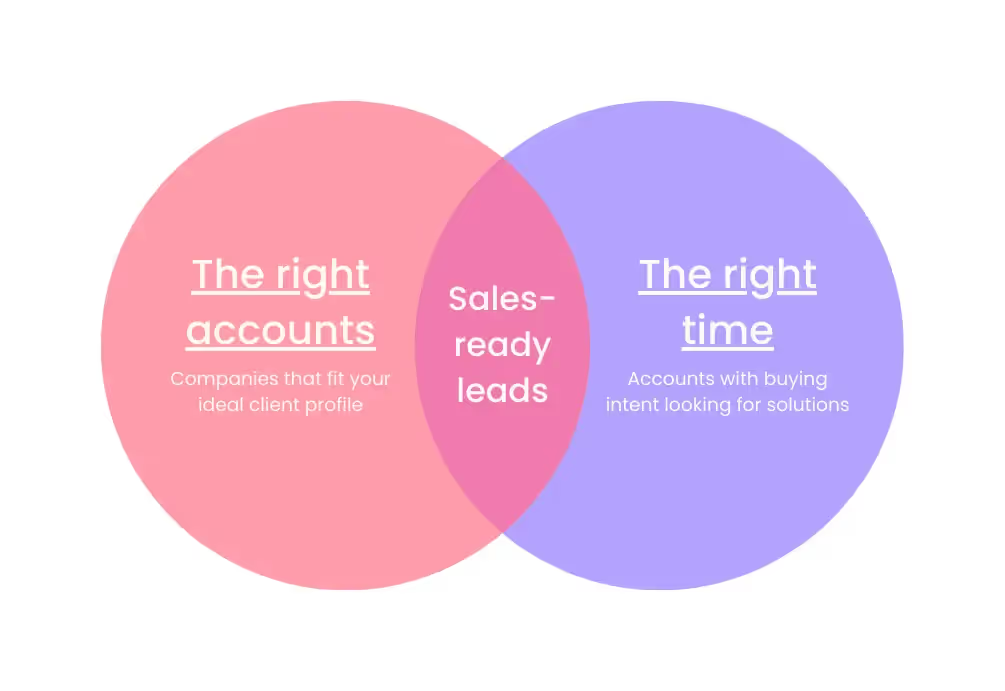
The following guide explores how to identify and target sales-ready accounts with the combined powers of Factors’ account identification and HubSpot webhooks. We first discuss how this integration works, before delving into a handful of use-cases.
How It Works: Pushing data back into HubSpot
Factors taps into industry-leading IP-lookup technology to identify up to 64% of anonymous companies visiting your website. This includes company names as well as firmographics such as geography, industry, employee headcount, revenue range and more.

In addition, Factors auto-tracks website activity and engagement with advanced analytics. This includes page views, button clicks, scroll-depth, account timelines, funnels and more.
With this information, users can filter the total set of anonymous traffic down to ICP accounts that have expressed buying intent:
- ICP criteria: Filter down traffic based on firmographics such as industry, headcount and revenue-range to identify accounts that fit your ideal client profile.
- Intent criteria: Filter down traffic based on intent signals such as high-intent page views such as pricing, time-spent on page, and percentage scroll-depth to identify sales-ready buyers.
In short, access a list of high-intent ICP accounts that are already visiting your website but are yet to convert.
Now, with webhooks and Zapier, it’s easier than ever to automatically push all this data from Factors into any other tool your team uses. This includes ad platforms, marketing automation platforms, and, in this case, HubSpot CRM.
How will this help? Rather than going after cold prospects with negligible chances of conversion, sales reps can view, segment, and target sales-ready accounts inside HubSpot. As we’ll see in the next section, this dramatically simplifies and improves targeted sales outreach.

Implementing Webhooks on Factors is easy as pie. See how here.
Use-cases: Making the most of your website traffic
1. Identify new business opportunities
Factors surfaces anonymous, high-intent companies visiting your website. As previously discussed, this data can be filtered down to high-fit, high-intent accounts.
Using webhooks, this data can be pushed from Factors into HubSpot. In other words, you can automatically create companies inside HubSpot for visiting companies that match your ICP and intent criteria.
For example, webhooks can be configured to create a new company when a visitor from a US-based software company with at least 250 employees is live on your website.
Here are a few more examples of what you can see inside your CRM with Factors:
- Accounts that visit a landing page through a search ad but fail to submit a form
- Software companies with at least 500 employees visiting high-intent pages like pricing
- US-based companies that have read through at least half a product comparison blog
Rather than relying on the 5% of website traffic that submits a form, teams can identify and target a deep new pool of potential pipeline — all within HubSpot. What’s more? Alerts can be relayed to sales reps in real-time through Slack or MS teams so they can immediately reach out to live prospects.

2. Stay on top of existing target accounts
In addition to recording new accounts visiting your website, Factors can be used to monitor and update data for target accounts that already exist within HubSpot.
For example, say an accounts clicks on a search ad, submits a demo form, but never schedules time on your calendar. While account's data is available in HubSpot, it can be tedious to track and update their actions post the demo form submission.
To solve for this, Factors can automatically update CRM properties based on trigger criterias when accounts return to your website. Let’s say that the same account is back reading a product alternatives blog or visiting the pricing page after a couple of weeks. This event can be updated within HubSpot, including their last active time.

Sales reps can be notified with real-time when high-intent events take place so as to be able to immediately reach out to accounts and improve the odds of conversion.
3. Accelerate deals with behavioral data
Certain marketing material may or may not be relevant depending on the audience in question. For example, an enterprise-level account may be especially interested in security compliance related content. An early-stage start-up, on the other hand, may find content around cost-effective pricing more appealing.
Factors can track how various types of companies are interacting with your website to understand what target accounts care about most. This data can be pushed back into HubSpot so sales reps can easily assess a prospect’s interactions, priorities and pain-points before jumping into a sales call.
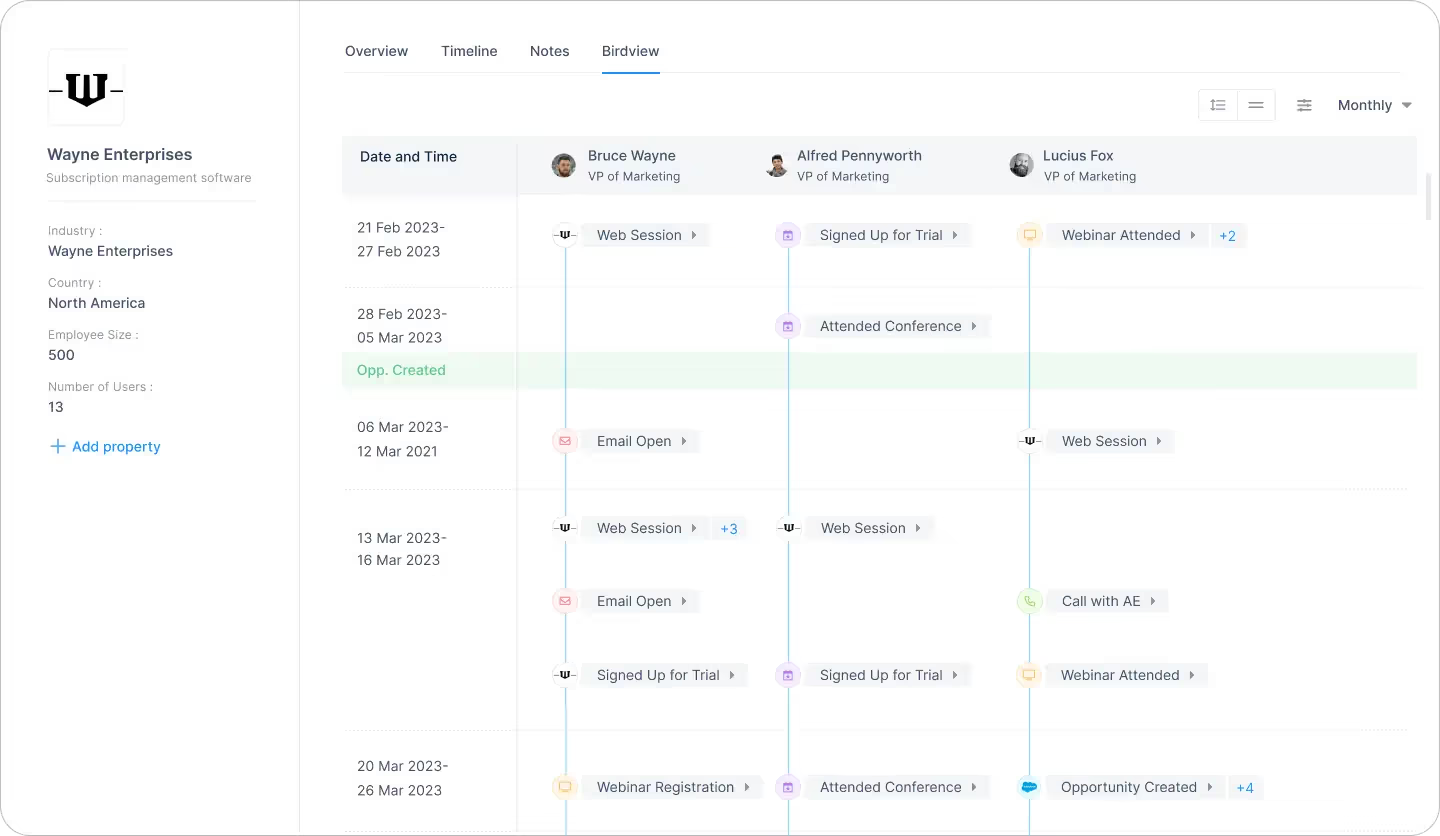
For one, sales reps can accelerate deals by personalizing the customer experience. For another, marketing teams can gauge what resonates best with the target audience and finetune content efforts accordingly.
4. Rekindle lost opportunities
Use Factors to track how prospects who have dropped off the funnel or former customers are returning to engage with your website. For instance, maybe an account that churned a couple of quarters ago is back interacting with a page that highlights a new feature release.
This may be an intent-signal that the account is reconsidering your product. It might be a good idea for sales reps to reach out and share some relevant information on what’s new. Of course, this doesn’t necessarily guarantee a conversion. But it’s far more effective than reaching out to an ice cold prospects.
This guide has covered a handful of ways in which pushing account data back into HubSpot can be helpful. Ultimately, the goal is to align account data with relevant stakeholders and technologies in order to:
- Drive intent-based sales outreach
- Refine ABM efforts and spends
- Optimize retargeting campaigns
There are countless other use-cases with account identification working in conjunction with CRMs, MAPs, and more. With webhooks, Factors can push valuable account data to nearly any platform on the planet. How you make the most of that data is really up to you — the possibilities are endless.
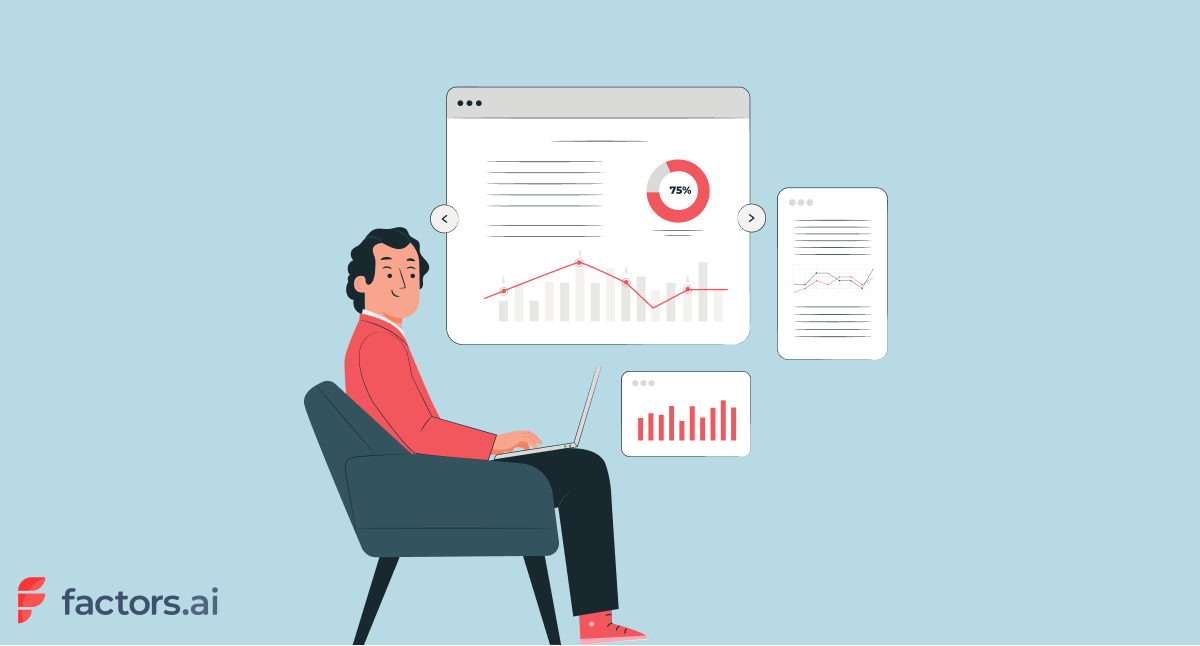
7 Benefits Of Marketing Analytics For Customer Experience
Mastering marketing analytics is key to any business that wants to become a brand.
Today, 73% of customers find user experience to be the most critical factor in making a purchase decision. And to give customers that experience, analytics is your ultimate weapon.
But can a business curate an experience worth remembering without any insight into its customers? The answer is a big NO.
Marketing and customer analytics provide valuable insights into customer behavior.
Let us understand why with these 7 Benefits of Marketing Analytics for Customer Experience.
7 Benefits of Marketing Analytics For Customer Experience

1. Better Customer Engagement
Marketing analytics is a reflection of customer behavior. If a customer likes what they see—they are more likely to engage. If they don’t, they’ll bounce off.
By analyzing customer data, teams can gain a deeper understanding of customer pain-points and preferences.
For example, enterprise level customers may be more interested in privacy-compliance while material around cost-effective plans may appeal more to smaller teams.
One can further gauge these preferences by testing hypotheses and running A/B tests.
Armed with this information, one aligns their sales and marketing team to create more targeted and effective marketing campaigns for a business, tailored specifically to the targeted customers and enterprises.
This personalisation is the benefits of marketing analytics, hence helping a brand become trustworthy and more valuable.
2. Reducing Churn Rate
Let’s understand Churn with an example. If 1000 visitors signed up for your services, but 50 of those stop doing business with you, your business has a Churn rate of 5%.
The churn rate is the percentage of customers who stop doing business.
By analyzing customer data, you can identify the factors that are causing customers to leave. This can be attributed to poor customer support, limited progress in the product road map, cost issues, or better alternatives being available in the market.
Whatever the case, marketing analytics helps pin-point where customers are coming from, and why they're leaving. Additionally, you can use this data to retarget your customers with the right message to regain them.
And because they already know about you, the conversion rate will be much higher and you’ll be able to earn loyal customers with minimum effort.
To do so, your business can identify common pain points—by monitoring customer feedback—and take proactive steps to gain benefits of marketing analytics for customer experience.
3. Increased Lifetime Value
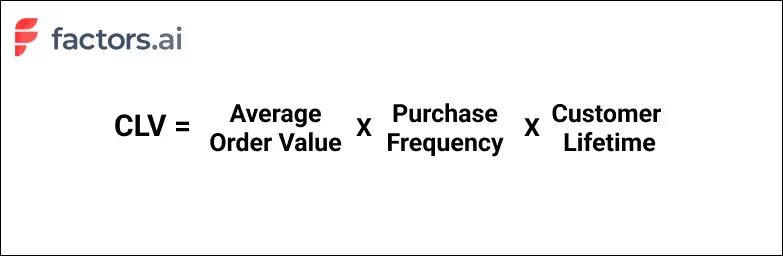
For any business, Customer Lifetime Value is one of the most important metrics. CLV is a metric that represents the total net value a customer brings to a business over the duration of their relationship.
And one of the many benefits of marketing analytics is, you can put systems in place that extend your customer loyalty.
One of the best examples of this insight in practice is Amazon Prime.
This loyalty program was started back in 2005 and has been one of the most important parts of the business today.
It has been reported that Prime customers spend $1400 per year on products, whereas non-Prime customers spend just $600 on average.
If a customer is likely to stay with the brand and make higher purchases—they surely are adding a lot more value. Hence helping businesses gain benefits of marketing analytics.
Additionally, since they are sticking with your brand, you don’t have to spend a lot on customer acquisition as well. Less spending and more earning equals better business.
4. Increased Customer Loyalty
Why do businesses offer discounts? Or create ungated content?
The answer to both these questions—and many more—is to gain customer loyalty.
It is one of the most important brand assets, which in today’s world holds a lot of value. This can be seen with brands like Supreme, Apple, and Coca-Cola.
Each one of such brands has developed a customer loyalty unique to their brand. They have gained benefits of marketing analytics for building brand experience.
It has not just happened on its own. All this has been a clear benefit of marketing analytics. Brands have used the tracked customer data using CRMs tools like ActiveCampaign and ActiveCampaign alternative to create unique experiences.
They use it to create ads, target customers, create UI, write email and form an overall experience for their customers. All that is to ensure, there are no leaks in the funnel.
5. Improved Customer Journey
In this whole list of the benefits of marketing analytics, we have talked about customer experience a lot.
But what will you see in your analytics exactly? You’ll see your customer journey.
You’ll see how a customer learns about your product, comes to your website, goes to the pricing page, and eventually signs up.
But what if you see that many customers who are visiting your pricing page are not signing up?
Your Customers might not send you a message about this, but they clearly are not seeing the right “value for money” proportion.
At this point, what you can try is—to improve your pricing page. This can be done by adding reviews, stats, small case studies, or examples on this page.
You’ll only be able to know how to improve customer journeys by tracking Marketing analytics and understanding what’s holding them back. And this understanding is one the main benefits of marketing analytics for customer experience.
Once all the friction is removed, the journey from social media engagement to sign-up would be much smoother and quicker.
6. Improved Customer Support
Customer support is a very important part of creating an effective experience for your customers.
It helps users be sure that your business is reliable and will help them solve any problem they might face.
But here’s a thing that you might not know. 86% of customers prefer to solve their problems on their own, rather than contacting customer support.
Leveraging social media channels like Instagram and Facebook, or WhatsApp for customer service, can offer efficient self-service options and quick responses, aligning with this customer preference.
If you are seeing a lot of “How-to’s” with your product—what your customer needs is a class on how to use your product effectively.
For this, you can use public platforms like Youtube, Vimeo etc. But if you are going to have a different class and session you might need tools like Teachable or Teachable alternatives for your business.
One of the core benefits of marketing analytics will only be uncovered once you start to understand the hurdles your customers are facing, and how to eliminate them.
7. Increased Referrals
Referrals are the result of having the best customer experience. If someone is recommending your service or product to someone else, that means they have trust in your brand.
And your business has gained this trust and this customer experience is understanding the benefits of marketing analytics.
Right from the social media post you shared to the pricing page you optimized—Marketing analytics have been your stepping stone.
And if you are not using this data, I am sure your business has a lot more potential than you think.
Key takeaways
For any entrepreneur that is looking to grow their business, and become a well-known brand among customers—the benefits of marketing analytics are limitless.
We all are aware of the story when Jeff Besoz updated the Amazon hardware page to make it easy for customers to buy the right screws.
All that was because of feedback he got from the customer. Now for most businesses, a customer might not even know what exactly will help them.
But if you can deliver something that matches their expectation of the experience—you and your business are on the right path of growth.
About the author
Divya is a marketer, nature lover & startup enthusiast. Founder of Unifiedist. She has an immaculate experience in GTM strategy & SEO. She always follows her instinct and travels with her Ikigai.

LinkedIn Intent Data: The Missing Ingredient in Your B2B Sales Strategy?
LinkedIn Intent Data: The Missing Ingredient in Your B2B Sales Strategy?
With over 900 million members across 200 countries and 4 out of 5 members driving business decisions, LinkedIn is a crucial platform for B2B sales and marketing teams. 97% of B2B marketers use LinkedIn for lead generation. But how can you ensure that your LinkedIn ads and marketing efforts are influencing conversions?
Enter LinkedIn intent data.
LinkedIn intent data shows if a prospect has interacted with or shown interest in your LinkedIn ads, allowing you to gauge their likelihood of converting. In this article, we'll discuss how this data can benefit both marketing and sales teams, and how Factors can help you make the most of this valuable information.
TL;DR
- LinkedIn intent data reveal prospects' level of interest and engagement with ads.
- It helps illuminate the "dark funnel" of hidden interactions and potential leads.
- Benefits include audience segmentation, ad optimization, retargeting, and lead scoring.
- Marketers can use intent data to optimize campaigns and improve targeting.
- Sales teams can use intent data to prioritize high-intent accounts and personalize outreach.
- Factors is a tool that integrates LinkedIn intent data with CRM data for deeper insights.
What Is LinkedIn Intent Data?
LinkedIn intent data is a crucial piece of information that reveals the level of interest and engagement prospects have with your LinkedIn ads. By analyzing this data, you can gain valuable insights into the buyer's journey and identify potential leads who are more likely to convert.
This information is vital in today's competitive B2B landscape, where understanding the preferences and needs of your target audience can significantly improve your sales and marketing efforts.
Why Is LinkedIn Intent Data Important?
In B2B, the buyer's journey is often complex and multifaceted. Prospects interact with various touchpoints before making a purchase decision. Unfortunately, not all of these interactions are visible or easily tracked, leading to the existence of a "dark funnel."
What is the dark funnel?
The dark funnel is part of the buyer's journey where prospects have been exposed to your LinkedIn ads, content, or other marketing materials but haven't directly engaged with them or converted immediately.
These “hidden” interactions can make it challenging to assess the true impact of your marketing efforts and identify valuable leads who may convert later in their journey.
How does Linkedin intent data help?
LinkedIn intent data illuminates the dark funnel by providing insights into prospects' level of interest and engagement with your ads, even if they haven't directly interacted with them. Here are a few ways in which it can help you gain a deeper understanding of the dark funnel:
- Segment your audience based on their intent data: By analyzing LinkedIn intent data, you can segment your audience into different categories based on their level of engagement and interest in your ads. This will help you create tailored account-based marketing campaigns that address the unique needs and preferences of each segment, increasing the chances of converting these prospects. For instance, if you run an e-learning platform, you can segment users who have engaged with your ads about coding courses and target them differently than users who’ve shown interest in a writing course.
- Optimize your ad creatives and targeting: Understanding the preferences of prospects within the dark funnel can help you optimize your LinkedIn ad creatives and targeting strategies to better resonate with your audience. Then, fine-tuning your ads based on intent data insights can improve the overall effectiveness of your marketing efforts. For example, if you find that prospects in the dark funnel are engaging more with video ads than image-based ads, you can allocate more budget to video ad campaigns and optimize targeting to reach more people likely to be interested in your product.
- Retarget potential leads: With this data at hand, you can retarget prospects with tailored content and offers. If a prospect has engaged with content about a particular product or service on your website, but didn't complete a purchase, you can retarget them with a special discount or offer, encouraging them to revisit your site and complete the transaction.
- Enhance lead scoring and prioritization: By incorporating intent data, you may find that a group of people have engaged heavily with your ads and content, but haven't reached out directly yet. For instance, if you're a B2B software company, your sales team can reach out to prospects who've shown a high level of engagement with specific features of the software. They can demo the tool while keeping the focus of the conversation on the feature of interest.
LinkedIn Intent Data For Marketers: Unlock The Potential Of Your Ads
As marketers, we aim to reach the right audience, deliver a message that resonates with them, and ultimately drive conversions. Linkedin buyer intent data is highly valuable in achieving these objectives and gaining valuable insights into audience engagement. With this, you can optimize your LinkedIn marketing strategy in several ways:
- Know if ads are reaching the intended audience: Linkedin’s intent data gives you insights into who is interacting with your ads. This information is then curated in an account-level format so your ABM teams can filter accounts with the highest interest in buying your product or service.
- Optimize LinkedIn ads based on engagement: Analyzing LinkedIn intent data can tell which ad formats, visuals, and copy resonate most with your audience. You can then make data-driven decisions to optimize your ads, boosting engagement and conversion rates.
- Know which kind of ad copy resonates with the audience: By examining the intent data from different ad variations, you can identify the messaging that best captures your audience's attention. This empowers you to tailor your ad copy and creatives to better appeal to your target audience, leading to more clicks, higher engagement, and ultimately, more conversions.
- Combine LinkedIn intent data with third-party intent data for a holistic approach: By integrating this data with third-party intent data (from sources like G2, TrustRadius, etc.), you can create a more comprehensive understanding of your prospects' needs throughout their buying journey. This enables you to deliver targeted, relevant content and ads that address their pain points and move them closer to conversion.
How To Use LinkedIn Buyer Intent To Improve Pipeline Velocity
Pipeline velocity is a key performance indicator (KPI) for sales teams, as it measures the speed at which leads move through the sales funnel and ultimately convert into customers. Leveraging LinkedIn buyer intent data can significantly improve pipeline velocity by helping you prioritize high-intent accounts, personalize outreach, and align sales and marketing efforts. Here's how:
- Prioritize high-intent accounts for account-based marketing (ABM): LinkedIn buyer intent data can help sales and account-based marketing (ABM) teams identify high-intent accounts, those that have shown significant interest in your LinkedIn ads, and are more likely to convert into customers. By prioritizing these high-intent accounts, your team can focus its efforts on the most promising leads, increasing the chances of closing deals and improving overall pipeline velocity.
- Use intent data and deanonymization to personalize outreach: Using LinkedIn buyer intent data, you can tailor your sales outreach to the specific needs and interests of your prospects. Adding Factors to the mix, you can also deanonymize website traffic and know about the company and industry that they work in. With this, you can gain deeper insights into their pain points, preferences, and points of friction. This allows your sales team to craft personalized messages that address these concerns and demonstrate the value of your product or service, helping to move prospects through the sales funnel more quickly.
- Align sales and marketing based on intent data: Regularly analyzing LinkedIn buyer intent data can help you identify trends and patterns in your prospects' behavior, allowing you to optimize your sales and marketing tactics accordingly. This is also good for reporting on the effectiveness of campaigns. For example, you may discover that certain types of content or ad formats resonate better with your target audience, leading to higher engagement and faster movement through the sales funnel.
- Add intent data into CRM and marketing automation platforms: Integrating LinkedIn buyer intent data into your CRM and marketing automation platforms can help automate lead scoring, segmentation, and nurturing efforts based on prospects' engagement and intent. This allows your sales and marketing teams to efficiently focus their efforts on high-intent leads, ultimately improving pipeline velocity.
- Enhance Account Scoring: Incorporating LinkedIn buyer intent data into your account scoring methodology can provide a more accurate assessment of a prospect's likelihood to convert. The combination of data from multiple sources allows your sales and marketing teams to better score leads. Add to that Factors’ account scoring features, and you can automate your prioritization process for the teams too.
Make The Most Of Your LinkedIn Intent Data with Factors
Leveraging buyer intent data from LinkedIn effectively can help you identify the users with the highest interest and modify your approach to better target the accounts. Factors enables you to maximize the potential of this data to drive results. Here's how:
1. Integrated data analysis

Factors helps combine Linkedin intent data with data from other sources, such as CRM platforms, ads, website, and more. This integrated approach enables you to gain a holistic understanding of your prospects' buyer journey, helping you identify high-intent accounts and optimize your marketing and sales strategies accordingly.
2. Visual user timelines for enhanced attribution
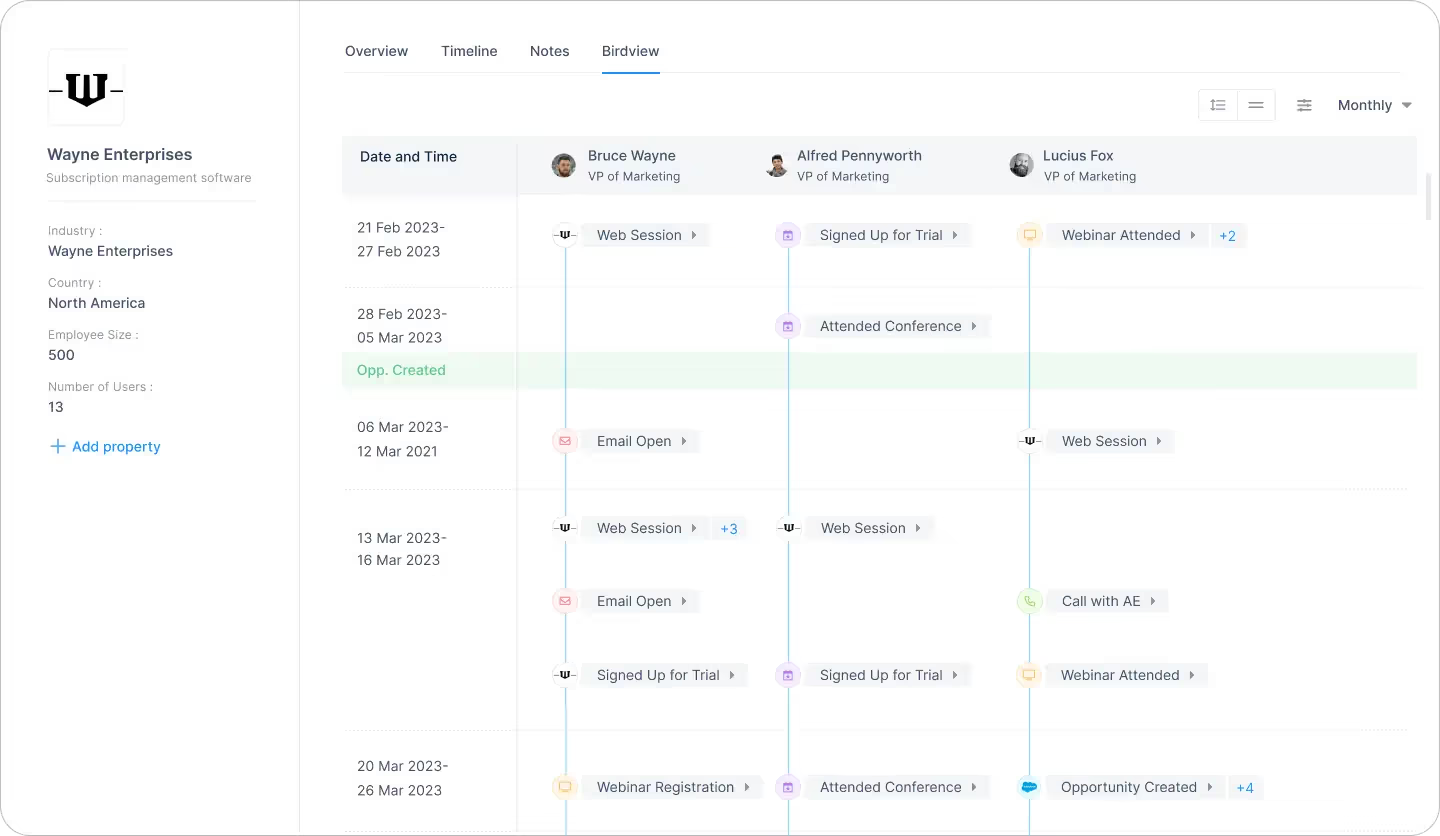
Factors offers a visual user timeline that lets you track and analyze your prospects' interactions with your LinkedIn ads over time. You can also view if the same user has interacted with your brand over other platforms or campaigns that you already track. This gives you a holistic view of an individual user's journey and engagement with your brand across multiple touchpoints.
3. Account intelligence
Factors' account intelligence capability allows users to identify anonymous companies visiting your website, along with their intent and firmographics. Your ABM teams can then personalize outreach by understanding their interactions with your content and ads to improve conversions.

4. Optimize ad engagement and performance
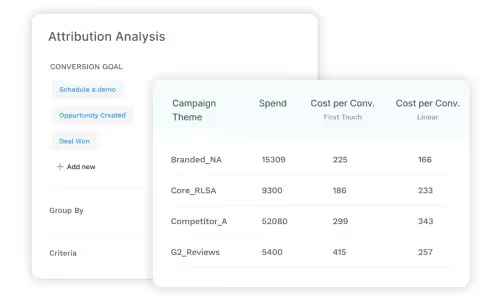
Factors uses Linkedin ad engagement data and audience insights to help you optimize your Linkedin ad campaigns. Knowing which ads resonate with your target audience and generate the most engagement can help you refine your campaigns to maximize returns and drive conversions. You can also uncover the ad formats, content types, and messaging that resonate best with your target audience and increase the likelihood of conversions.
5. Account scoring

Factors' account scoring feature helps prioritize prospects based on their engagement and intent, enabling sales teams to focus their efforts on high-value targets. By combining intent data with firmographics, technographics, and engagement history, you can create a comprehensive account score that helps your sales team prioritize their efforts and focus on the most promising opportunities.
FAQs
1. How does LinkedIn calculate buyer intent?
LinkedIn calculates buyer intent by analyzing user engagement with your company's ads, content, and other interactions on the platform. This includes factors such as clicks, likes, comments, shares, and time spent viewing your content. By tracking these interactions, LinkedIn can identify which users are genuinely interested in your products or services, signaling potential buyer intent.
2. How can you use intent data for sales?
Intent data can be used by sales teams to prioritize high-intent accounts, personalize outreach efforts, align sales and marketing initiatives, optimize sales tactics, and enhance account scoring. By leveraging intent data, sales teams can focus their resources on the most promising leads, creating more targeted and effective sales strategies that drive revenue growth and improve pipeline velocity.
Bring the Power of LinkedIn Buyer Intent Data to B2B Sales
Leveraging LinkedIn buyer intent data can revolutionize your sales and marketing efforts, enabling you to prioritize high-intent accounts, personalize outreach, and align your teams for maximum impact.
By using powerful tools like Factors, you can gain a comprehensive view of buyer journeys and make data-driven decisions to boost pipeline velocity and drive revenue growth. Don't miss out on valuable opportunities – uncover the potential of LinkedIn buyer intent data and elevate your B2B sales strategy today.
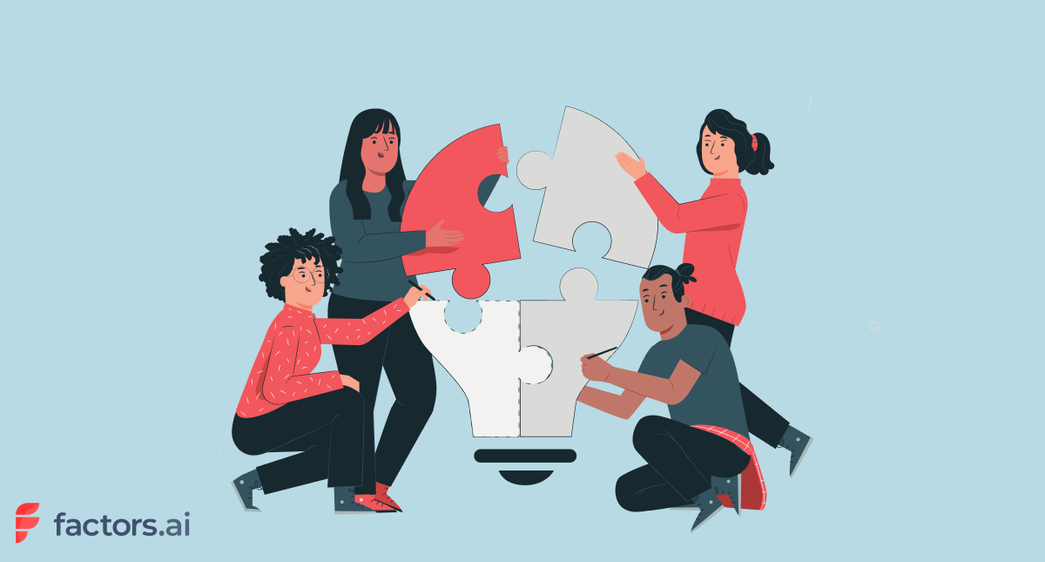
Account-Based Marketing Team Structure: Key Roles and Responsibilities to Drive Success
Account-based marketing (ABM) is unlike traditional marketing. Instead of trying to reach the masses, you focus on a small set of high-value accounts.
The ABM team crafts individually tailored content, advertisements, and emails for the target accounts, increasing the likelihood of conversion. For instance, consider a company that sells cybersecurity solutions to financial institutions. The target accounts are large banks and credit unions looking to upgrade their cybersecurity measures.
The ABM team creates tailored content such as case studies, whitepapers, and infographics. They also design advertisements highlighting the revenue losses from security breaches. All the content is tailor specifically for the financial industry, and sometimes even for specific companies.
This targeted approach makes ABM a powerful strategy. Businesses that used ABM strategies saw revenue growth of 208% and an average increase of 171% in their annual contract values.

But you need a strong account-based marketing team structure to succeed. Without a proper team, even the most ambitious ABM strategy can quickly fall apart.
That’s why it’s important to know the key players and qualities of a good ABM team member before you begin structuring your ABM department.
TL;DR:
- Account-based marketing (ABM) focuses on high-value accounts, requiring a well-structured team and diverse skill sets
- Key team members include C-level executives, data analysts, strategists, designers, and content creators
- CEO, CMO, and CRO provide strategic direction, align ABM with company goals, and drive revenue growth
- Operations, Marketing, and Sales Managers oversee and execute various aspects of ABM campaigns
- Execution-based roles include Performance Marketers, Graphic Designers, Content Marketing and Strategy, Social Media Marketers, and Copywriters
- Proper team structure is critical for ABM success. It requires collaboration, strategic thinking, adaptability, and strong communication skills
- Tools like Factors.ai can optimize ABM efforts by providing insights into customer journeys, visitor tracking, and marketing ROI optimization
Account-Based Marketing Team Structure

The account-based marketing team brings together people of diverse skill sets and varying levels of expertise to come together with a focused vision.
C-Suite and Directors
The C-Suite and Directors in the ABM team have higher-level access to company information and the long-term vision to align the team towards a singular goal.
Chief Executive Office (CEO)
Before any ABM campaign is planned out, the team needs to understand the long-term vision of the company. That’s where a CEO comes into play. With the top level view of the company, the CEO can assist the ABM team plan things out, provide feedback on strategies, and assist with connecting the team to high-value accounts through their networks.
Some of the key responsibilities of the CEO in terms of the ABM team are:
- Setting the company's vision and long-term strategy
- Providing leadership and guidance to the executive team
- Building and managing relationships with key stakeholders
- Representing the company to the public and media
- Work with stakeholders for account scoring
Chief Marketing Officer (CMO)
The CMO has a critical role in the ABM team. This person helps define the strategy and keeps the ABM team aligned to the company’s goals at all times. The responsibilities may vary, but a CMO is generally involved in:
- Providing strategic direction and guidance for the ABM program
- Aligning ABM initiatives with the company's overall marketing strategy
- Collaborating with the sales team to identify target accounts and prioritize outreach efforts
- Ensuring that the ABM team has the necessary resources and tools to execute campaigns effectively
Chief Revenue Officer (CRO)
The CRO manages all things revenue and has the highest level access to the company’s inflow and outflow. A CRO can help the ABM team to:
- Bring the sales and marketing teams together to create a cohesive ABM strategy
- Ensure high-quality leads and revenue growth through the ABM program
- Approve budgets to execute campaigns as and when required
- Measure and analyzing the ROI of campaigns
- Collaborate with the marketing team to help refine the ABM strategy over time
Sales Directors
Sales directors are responsible for driving revenue growth by managing the sales team and maintaining relationships with key clients. The sales directors might be involved in:
- Collaborating with the marketing team to identify target accounts and prioritize outreach efforts
- Providing feedback on the effectiveness of ABM campaigns in generating leads and driving revenue
- Helping to refine the ABM strategy over time based on sales team feedback
- Ensuring that the sales team is aligned with the ABM program and has the necessary resources to engage with target accounts effectively.
Managerial Roles in ABM
The success of an Account-Based Marketing (ABM) campaign is heavily dependent on the leadership and management of the team. Managers oversee and help with executing various aspects of ABM campaigns.
Operations Manager
The Operations Manager oversees ABM campaigns from planning to execution. They ensure that all tasks are completed on time and that the team works efficiently. The ops manager also helps the ABM team manage the budget and execute tasks cost-effectively.
Some of the key responsibilities of the Operations Manager in ABM include:
- Overseeing the development of the ABM strategy and ensuring it aligns with the company's overall goals
- Managing the budget for the ABM campaign and ensuring that expenses are within the allocated budget
- Setting up systems and processes to track the progress of the ABM campaign
- Collaborating with the Marketing and Sales team to ensure that the campaign is effective in generating leads and revenue
- Reporting on the progress of the ABM campaign to senior management
Generally, the operations manager needs to be on top of things to ensure proper execution of the campaigns.
Depending on the org structure in the company, operations manager may also keep track of the key ABM metrics like customer acquisition, customer retention, and customer engagement.
This can help determine whether the current marketing strategies are effective and whether they need to be modified.
Marketing Manager
The Marketing Manager is responsible for the creative aspects of the ABM campaign, such as developing the messaging and designing the creatives. They work closely with the Operations Manager to ensure that the campaign is executed according to plan. Some of the key responsibilities of the Marketing Manager in ABM include:
- Developing the messaging and creatives for the ABM campaign
- Identifying the right channels to reach the target accounts
- Developing and executing marketing campaigns that align with the ABM strategy
- Measuring the effectiveness of marketing campaigns and making necessary adjustments
- Collaborating with the Sales team to ensure that marketing efforts are aligned with sales objectives
Since a major part of the marketing manager’s role is understanding analytics and data, they can greatly benefit from marketing analytics tools like Google Analytics, Factors.ai, and Microsoft Clarity.
These tools can help measure the performance of marketing campaigns, track visitors and engagement, perform revenue attribution, and identify areas for improvement.
Sales Manager
The Sales Manager is responsible for working with the Sales team to ensure that the ABM campaign is generating leads and revenue. They work closely with the Operations and Marketing Managers to ensure that the campaign is executed smoothly.
Some of the key responsibilities of the Sales Manager in ABM include:
- Collaborating with the Marketing team to identify high-value accounts
- Identifying decision-makers and key contacts within the target accounts
- Developing and executing a personalized outreach strategy for each account
- Reporting on the progress of the ABM campaign to senior management
- Nurturing relationships with key clients and ensuring their needs are met
Sales managers can also choose to employ a conversational ABM strategy to improve the sales team output. This strategy uses chatbots as the first point of contact, helping sales teams filter clients and improve conversions.
Strategy and Execution-Based Roles
While the senior-level team members provide strategic direction, the execution-based roles do the groundwork for ABM campaigns.
Performance Marketers
Performance marketers are responsible for creating and executing paid advertising campaigns. They come up with strategies to target the right audience, work with graphic designers to design ads, and monitor campaigns’ performance to optimize results. The responsibilities of performance marketers include:
- Creating the target audience and segmenting for better targeting
- Keeping track of campaign performance metrics using analytics tools like Factors and Google Analytics
- Collaborating with designers, content strategists, and copywriters to design and create ad copy and landing pages
Graphic Designers
Graphic designers play a crucial role in creating personalized and tailored designs for the ABM campaigns. But generic designs will fail to meet the standards here.
ABM designs need to capture the attention of your target audience and make a lasting impact.
Graphic designers must deliver their highest quality work, bringing creativity and innovation to the table. The designers must also have a deep understanding of the target account's preferences and expectations to truly resonate and drive engagement.
Here are some key responsibilities and qualities of a graphic designer in an ABM team:
- Collaborate with the marketing and strategy teams to create designs that resonate with target accounts
- Craft graphics for various marketing materials, such as display ads, social media posts, landing pages, and email campaigns
- Ensure that all visual elements are consistent with the company's branding and visual identity guidelines
- Optimize and repurpose graphic content for use on different social media platforms
Content Marketing and Strategy
Content is an important part of any ABM campaign. For instance, the content strategy team begins identifying topics that are important to your target audience.
The content marketing team then creates blog posts, whitepapers, and case studies around the topics to rank on search engines and be shared with the target accounts.
They may also collaborate with the sales team to identify content gaps and create additional content that speaks to the pain points of target accounts.
Some of the major responsibilities of the content marketing and strategy team may include:
- Conducting keyword research to optimize content for SEO
- Developing content that speaks to the pain points of target accounts
- Creating a content calendar to ensure consistency in messaging
- Developing and executing on a social media strategy to promote content
- Measuring and analyzing the performance of content to make data-driven decisions
Social Media Marketers
Social media marketers are responsible for ensuring regular engagement with the target accounts. They mould the social presence in a way that the target accounts find value in following your company profile—thus giving you direct access to these accounts. The responsibilities of social media marketers include:
- Creating and managing social media accounts
- Developing social media strategies that align with the ABM campaign's objectives
- Creating social media content that resonates with the target audience
- Engaging with the target audience on social media channels
Copywriters
Copywriters are responsible for creating compelling copy that resonates with the target audience. They work closely with content strategists to ensure that the copy aligns with the ABM campaign's objectives. The responsibilities of copywriters include:
- Creating copy for ad campaigns, landing pages, and other marketing materials
- Collaborating with content strategists to ensure that the copy aligns with the ABM campaign's objectives
- Conducting research to identify the pain points of the target audience
- Writing compelling copy that resonates with the target audience
Why is team structure important for ABM?
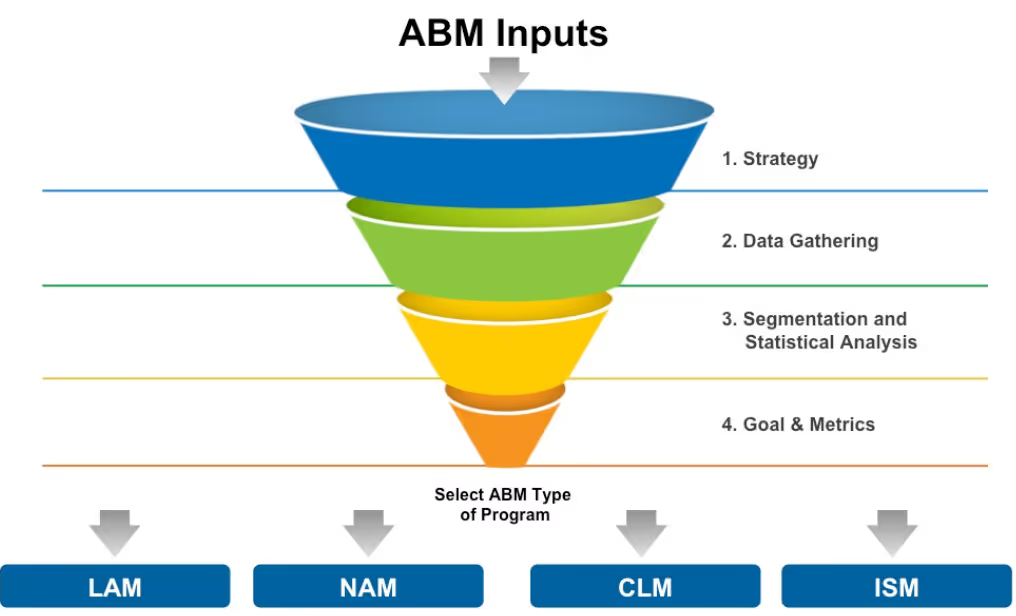
The process of an ABM campaign goes from designing the strategy, to gathering data and analyzing it, and finally executing the campaign based on the findings. But because of the highly personalized nature, account based marketing involves stakeholders from multiple teams for insights and feedback.
ABM teams need a lot of ad-hoc decision-making and creativity, so everyone on the team works towards a common goal, communicates effectively, and supports each other.
This is why proper team structure is critical to the success of ABM campaigns. It allows for seamless integration of strategies and effective collaboration among team members.
Apart from the basic understanding of the role and being able to collaborate with a diverse set of individuals, here are a few qualities of a great ABM team member:
- Use data and analytics to guide decisions and actions
- Ability to find creative solutions to challenges
- Adapt to changing situations and priorities
Achieve ABM Success With a Strong Team
A strong and effective team is crucial to the success of any ABM initiative. With the right mix of talent, expertise, and collaboration, your ABM team can unlock the full potential of your marketing efforts and drive meaningful results.
But to truly take your ABM to the next level, you need the right tools and technologies at your disposal. That's where Factors.ai comes into play. It offers deep insights into customer journeys, anonymous visitor tracking, and marketing ROI optimization, helping you to identify sales-ready accounts, automate analytics, and prove the revenue impact of every touchpoint.
With Factors, you can reduce your CAC, improve ROI, and accelerate revenue growth seamlessly. Schedule a demo today and see for yourself how Factors can transform your ABM approach and drive more pipeline with less spend.
FAQs
1. What qualities should I look for when building my ABM team?
Here are some of the qualities to look for in an ABM team member.
- Strong collaboration skills
- Strategic thinking.
- Analytical mindset
- Adaptability for constantly changing environment
- Creativity
- Strong communication skills
2. How do I create an ABM team?
Creating an ABM team involves understanding your end goals and finding people to fill the talent and skill gaps within your marketing and sales teams. However, here are the general steps to build your ABM team.
- Establish the end results you want to achieve with an ABM team
- Based on the goals, identify what skills and expertise is needed for your marketing team. This could include account management, data analysis, content creation, and project management
- Hire people with the required skill sets and establish clear roles and responsibilities for each of the new team members
- Create an open environment for the team to collaborate with the stakeholders as an when required for the successful execution of your ABM campaigns

5 Leadfeeder Alternatives For Website Visitor Identification [2024 Dealfront Update]
It’s official. Leadfeeder has joined forces with Echobot to form Dealfront — a Europe-centric go-to-market platform. As a result of this merger, Leadfeeder’s pricing plans have increased to start at $215+ per month with limited seats and data.
Several Leadfeeder users are looking for comparable sales intelligence alternatives to help identify and track anonymous website visitors.
If you’re in the same boat, or are simply considering your options, this is the right place for you.
The following article explores 5 comprehensive Leadfeeder alternatives to help your team make a seamless switch. We'll highlight key features, benefits, and drawbacks with each Leadfeeder alternative.
By the end of this article, we’d like to help you find an equally effective, if not better, alternative to your current visitor identification tool.
Looking to learn more about visitor identification? Check out these further readings:
Leadfeeder Alternatives For Website Visitor Identification
1. Factors.ai
Leading off our list is Factors.ai. Factors is unique in that it’s originally built upon strong analytics and attribution foundations. A recent partnership with 6sense, a leading enterprise-grade ABM platform, has propelled Factors into becoming a rising star in the visitor identification software space.
With this collaboration, Factors delivers industry-leading visitor identification, firmographics, and account timelines along with its existing ABM analytics and multi-touch attribution capabilities.
Factors.ai Key Features
Here’s a brief breakdown of Factors.ai’s key features
1. Account Identification
Of course, every tool featured on a list of Leadfeeder alternatives must be capable of identifying and tracking accounts visiting the website. Factors is no exception to this. In fact, as a result of its collaboration with 6sense, Factors taps into enterprise-grade IP data to identify up to 64% of anonymous companies — including firmographics (employee headcount, industry, location, etc) and website activity (page visits, time spent, scroll-depth, etc).
Factors also provides real-time Slack alerts to help teams stay on top of high-intent visitors and strike while the iron’s still hot. These alerts can be configured to go off based on specific firmographic features and website behavior — so you can target ICP companies visiting your site.

2. Account Scoring & Timelines
Factors integrates with campaigns, website, and CRM data to provide cross-channel account scoring and full-funnel timelines across the customer journey. With this feature, users can see exactly what touchpoints (ads, blogs, emails, webpages, etc) are influencing accounts to progress from top-of-the-funnel visitors to paying customers. Users can identify and prioritize high-intent accounts for focused outreach and re-marketing efforts
3. Advanced Analytics:
Unlike other solutions on this list (including Leadfeeder), Factors provides a wide range of analytics and attribution capabilities in addition to visitor identification. A few advanced analytics features include:
- ABM analytics - Integrates with ad platforms, CRMs, CDPs and more to unify reporting and support campaign analytics, website analytics, funnels, and more at an account level.
- Path analysis - View aggregate user behavior and identify points of inflection in conversions and drop-offs along the customer journey
- Multi-touch attribution - Connect the dots between go-to-market initiatives and pipeline, optimize resource allocations across campaigns, and prove marketing ROI with a wide range of multi-touch attribution models.

{{CTA_BANNER}}
Benefits of Factors.ai
Here are a few reasons why you may prefer Factors.ai to other tools:
1. Data accuracy: As previously mentioned, Factors taps into 6sense’s extensive database to provide industry-leading website visitor identification. In fact, rigorous testing with a sample data set of over 20,000 domains reveals that Factors identifies upto 27% more accounts than the closest alternatives — including established brands such as Clearbit and Demandbase.

2. Granular metrics: Given Factors’ roots in account analytics, it’s capable of reporting granular metrics and events as compared to other visitor identification alternatives. A few of these include:
- % scroll-depth
- Auto-button captures
- Cursor engagement
- Page time spent
3. Scoring & Alerts: Not all your website visitors are immediately read-to-buy. Some are further along the funnel than others. According, Factors supports custom account scoring models so you can qualify and be alerted to high-intent, ICP accounts engaging with your company in real-time.
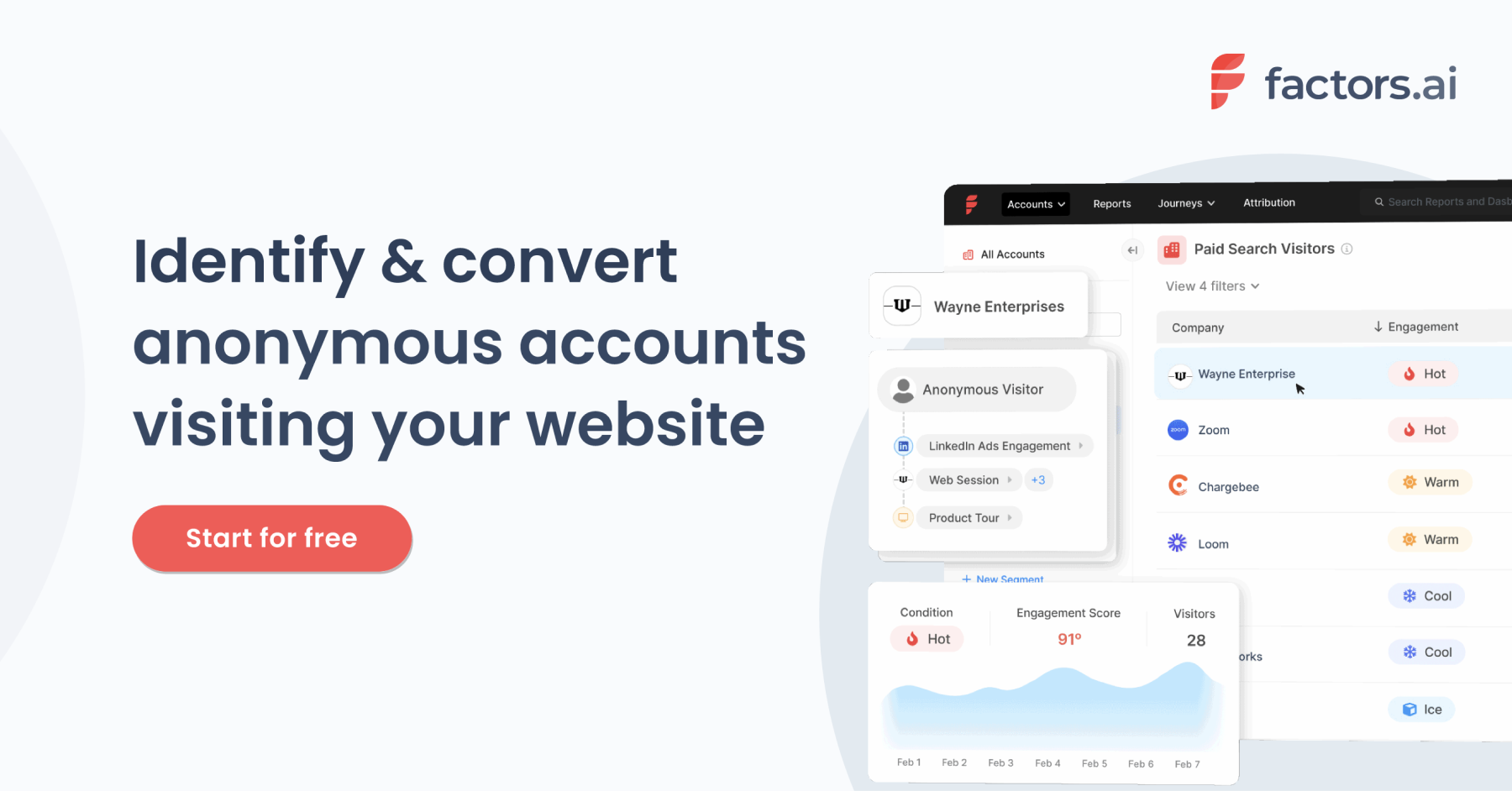
Drawbacks of Factors.ai
Contact-level enrichment - No privacy-compliant software can identify exactly which individual visited a website without having a visitor explicitly submit a contact form. That being said, the majority of tools on this list can source a list of ideal prospects to reach out to from the companies they’ve identified.
At the moment, Factors cannot provide this information natively. Instead, users can find contact information on third party tools such as Zoominfo or Apollo.
Factors.ai Pricing
Learn more about pricing here: factors.ai/pricing
Curious to see Factors in action? Book a personalized demo here.
{{CTA_BANNER}}
2. Albacross
Next up, we have Albracross. Albracross is one of the leading visitor identification and intent data companies in Europe. The Sweden-based platform works with over 10,000 companies to provide customer data enrichment, sales alerts, and intent signals.

Key Features
1. Account Identification: Like the other tools on this list, Albacross identifies anonymous accounts, firmographic information and visitor intent. Albacross features one of the largest proprietary first-party databases in the world.
2. Personalization: Albacross natively integrates with popular personalization tools such as Optimizely and VWO to customize website content based on who’s visiting the site. This is a powerful feature for product marketers looking to identify visitors and tailor messaging based on their profiles.
3. Display ads: A nifty feature offered by Albacross is the ability to launch and monitor display ads within the platform itself. The software partners with several publicists such as The New York Times and Daily Mail to distribute account-level targeted ads.
Benefits of Albacross
Here are a few reasons why you may prefer Albacross to other tools:
1. Experimenting: Given that Albacross is one of the few Leadfeeder alternatives to integrate with personalization tools, it offers the unique benefit for product marketers to experiment and run A/B tests in conjunction with visitor identification and intent data.
2. Customer success: Several reviews rave about Albacross’s stellar customer success management. Given that Albacross is considered to be an involved, enterprise-level tool, it’s essential to have this level of support to get the most value out of the product.

Drawbacks of Albacross:
Here are a few reasons you may consider other tools over Albacross:
1. Rigid firmographics and filters - Albacross seems to lack agility when it comes to filters and breakdowns. Reviews reveal that it’s currently not possible to filter identified companies based on firmographics such as name or size. As a result, users seem to find sorting and reporting somewhat challenging.

2. Buggy integrations: Multiple reviews claim that Albacross’s integrations, especially with CRMs like Salesforce, could do with some work. Given that visitor identification is primarily used to support ABM, this can be a major drawback to B2B teams. Tools like Zapier may be used to smoothen out workflows for now.

3. Lead Forensics
Founded in 2009, Lead Forensics is the oldest, most established website visitor identification software in this list. They own a sizable database of over 1.4 billion IP addresses, adding up to 55 millions contacts every year.
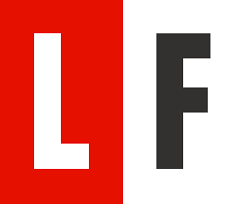
Key Features
Lead Forensics doesn’t have too many bells and whistles as compared to the other tools on this list. Its primary features are limited to visitor identification and contact data.
1. Account identification: Like Leadfeeder, Factors, and Albacross, Lead Forensics identifies anonymous businesses visiting your website by connecting the dots between IP addresses and company names. In the case of multinational companies, Lead Forensics is capable of showing which specific office is interacting with your website. This is handy for businesses looking to sell to large corporations.
2. Contact data: Lead Forensics also helps teams identify key contacts within companies visiting your website to assist with retargeting and outbound reachout. Here’s a brief summary of the kind of details that Lead Forensics can provide:
- Business name
- Contact details of key decision makers
- Telephone, email and LinkedIn details
- Demographics
- Search behavior
- Financial data

Benefits of Lead Forensics
A unique benefit with Lead Forensics is its mobile application. With their “Essential” plan, users can have trigger reports sent directly to their phones.
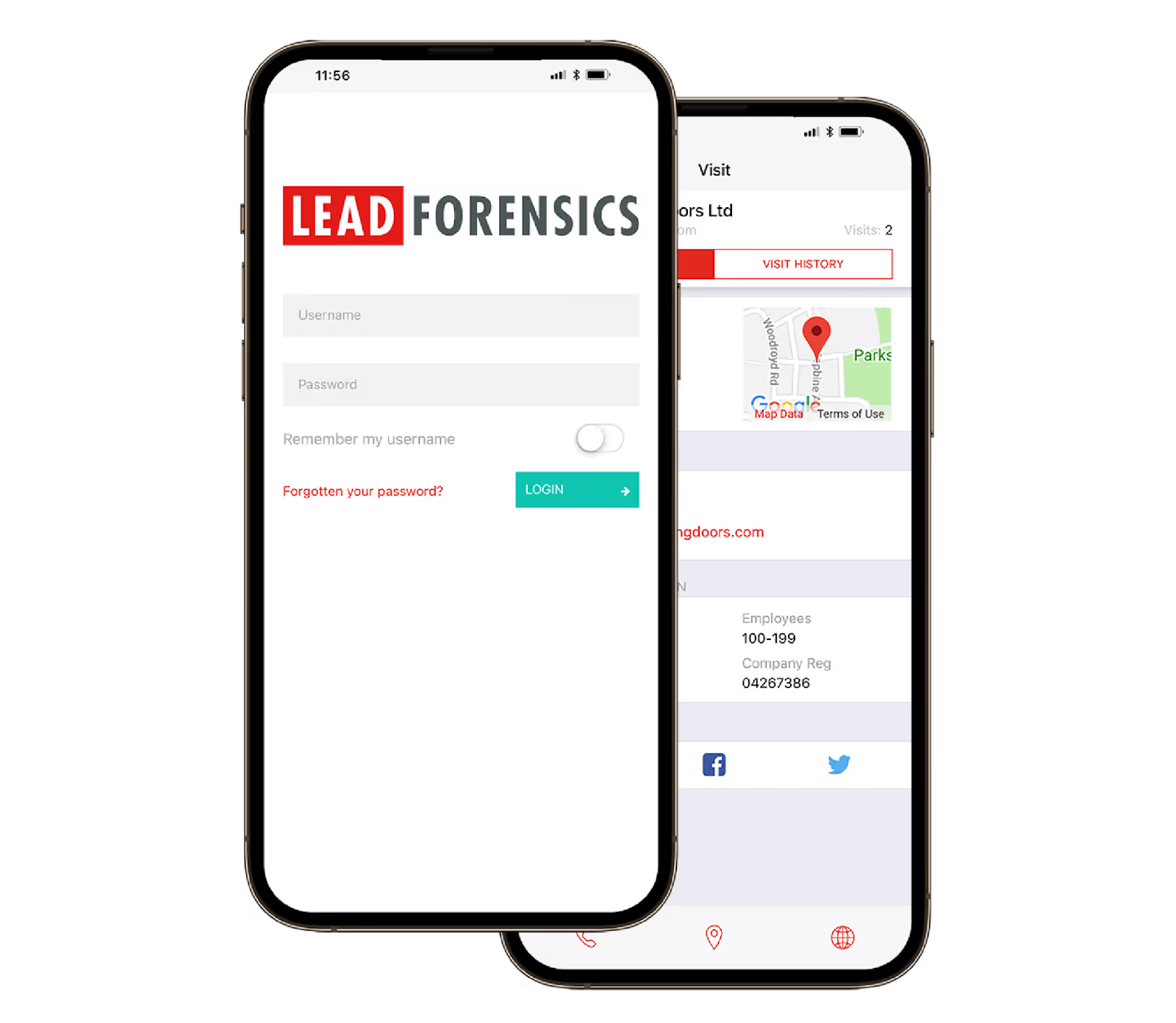
Drawbacks of Lead Forensics
Here are a few reasons you may consider other tools over Lead Forensics:
1. Poor UI - Lead Forensics is one of the oldest visitor identification software around. While experience is generally a positive sign, several users complain about Lead Forensics’ UI remaining outdated and unintuitive. Users find the tool to have limited functionality in terms of ad-hoc analysis, dashboards, and filters as well.
2. Pricing - While Lead Forensics does not openly reveal its pricing plans, it’s generally considered to be on the more expensive side. While this may not be a barrier for larger, enterprise-level companies, pricing may be prohibitive for small to mid-market start ups — especially when there are cost-effective alternatives available.

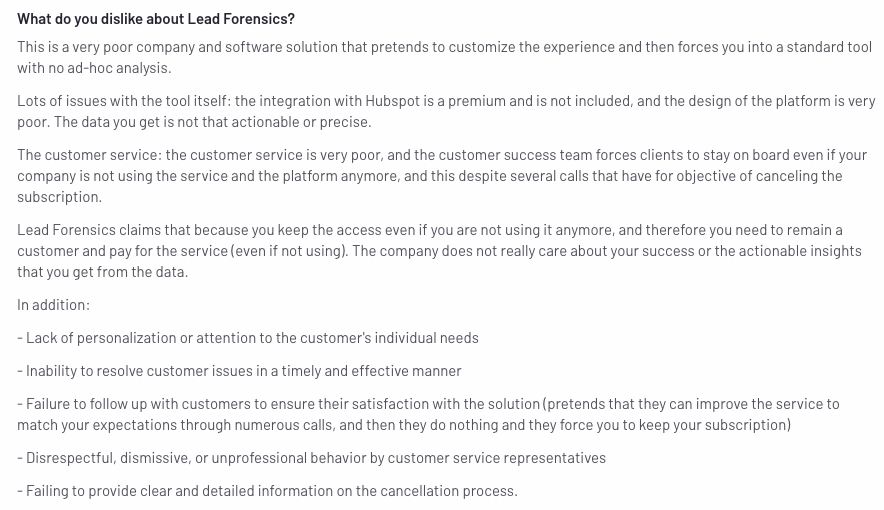
4. Clearbit
Fourth in our list is Clearbit. Clearbit is another well-established B2B data activation platform that offers a wide suite of products within its platform.
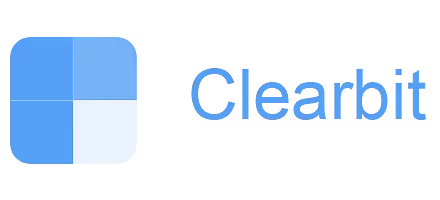
Key Features
Clearbit is divided into three broad products:
1. Reveal - Clearbit identifies anonymous website visitors using IP-lookup. Given that every tool on this list does this, data accuracy and pricing are two important considerations when differentiating between alternatives.
2. Enrichment - Clearbit also provides firmographics from over 250 data sources. This includes technologies, headcount, revenue, location, contact information, and more.
3. Capture - like Leadfeeder Contacts, identifies best-fit contacts from companies visiting your website to reach out to with retargeting campaigns or outbound efforts
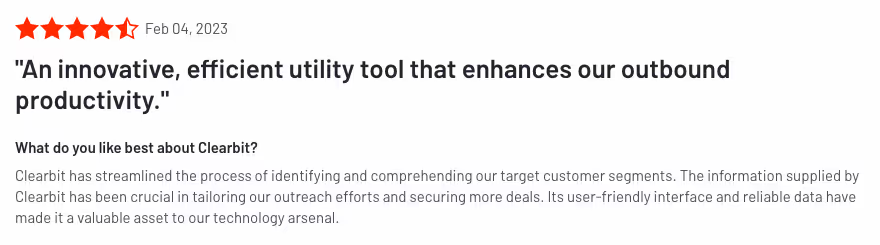
Benefits of Clearbit
1. Free plan: While Clearbit’s paid plans are relatively expensive, they offer a nifty free plan to de-anonymize anonymous traffic. That being said, the free plan is quite limiting as users can enrich only 50 domains a month. This becomes an issue as website traffic starts to scale over time. Still, it’s free — so who can complain?
Here’s a breakdown of what Clearbit’s free plan offers:
- Identify site traffic
- Calculate total addressable market size
- Determine ideal client profile
- Enrich domains and emails (up to 50 per month)
- Find email addresses (up to 100 per month)
2. Smart chat: Smart chat is a handy feature for sales reps to connect with high-value, high-intent visitors with personalized conversational ABM while visitors are live on the site. Chat prompts can be triggered based on visitor characteristics and behavior such as industry, page views, headcount, etc.
Drawbacks of Clearbit
1. Pricing: Clearbit does not reveal pricing details on its website. But several user reviews suggest that pricing is on the higher side for early to mid-stage startups.

2. Customer support: Clearbit works with a wide range of clients from early-stage startups to enterprise-level companies. Users from the former seem to face issues when it comes to customer support, claiming that it’s often slow and inadequate.

5. Visitor Queue
The final option on our list is Visitor Queue. Visitor Queue is a Canada-based visitor identification and personalization platform that identifies the name, contact detail and user data of accounts visiting your website.

Key Features
1. Visitor identification: What separates Visitor Queue from the rest of the tools on this list is its ability to aggregate company social profiles in addition to standard firmographics and contact information.
2. Personalization: Like Albracross, Visitor Queue allows users to personalize their website based on configurable criterias such as industry, source/medium, location, and more. This helps product marketers differentiate their brand from competitors and experiment with different messaging to improve conversions.

Benefits of Visitor Queue
1. Unlimited users - Most tools on this list (excluding Factors.ai) limit the number of seats per account. Given that visitor identification is used by sales, marketing, and customer success teams, it’s valuable to have an unlimited number of users with Visitor Queue.
2. Pricing - Visitor Queue is a more affordable option as compared to the likes of Clearbit and Lead Forensics with prices starting at just $31/month to identify 100 companies per month. This makes it a good option for early-stage teams that are working with tight budgets.
Drawbacks of Visitor Queue
1. Rigid reporting - While Visitor Queue does well at the basics, users complain about its restrictive UI when it comes to ad hoc reports, filters, and dashboards. As a company starts to grow, its requirements for tailor-made reporting do too. Visitor Queue wouldn’t back a good fit for custom usage.

2. Data issues - User reviews complain about data inaccuracies with Visitor Queue. While no tool is perfect, Visitor Queue seems to lag behind industry-standards in terms of accurately identifying visitors. While it’s great to have an inexpensive alternative, a visitor identification tool won’t be of much value unless it identifies the right visitors.

And there you have it! A brief summary of 5 comprehensive Leadfeeder alternatives to help you identify anonymous website visitors. Each of these tools have their pros and cons — and one is likely to be better suited to your needs than the others.
Let’s make the decision easier for you:
- If pricing and account-level data accuracy are priorities, Factors.ai is likely the best option.
- If the ability to personalize your website based on who’s visiting your site is what you’re looking for, Albacross and Visitor Queue are two great options.
- If you’re a larger enterprise without budget constraints, Clearbit could be the right option.
Factors.ai identifies up to 64% of anonymous visitors — the highest match rate in the industry. Looking to learn more about how Factors can help? Book a personalized demo here!
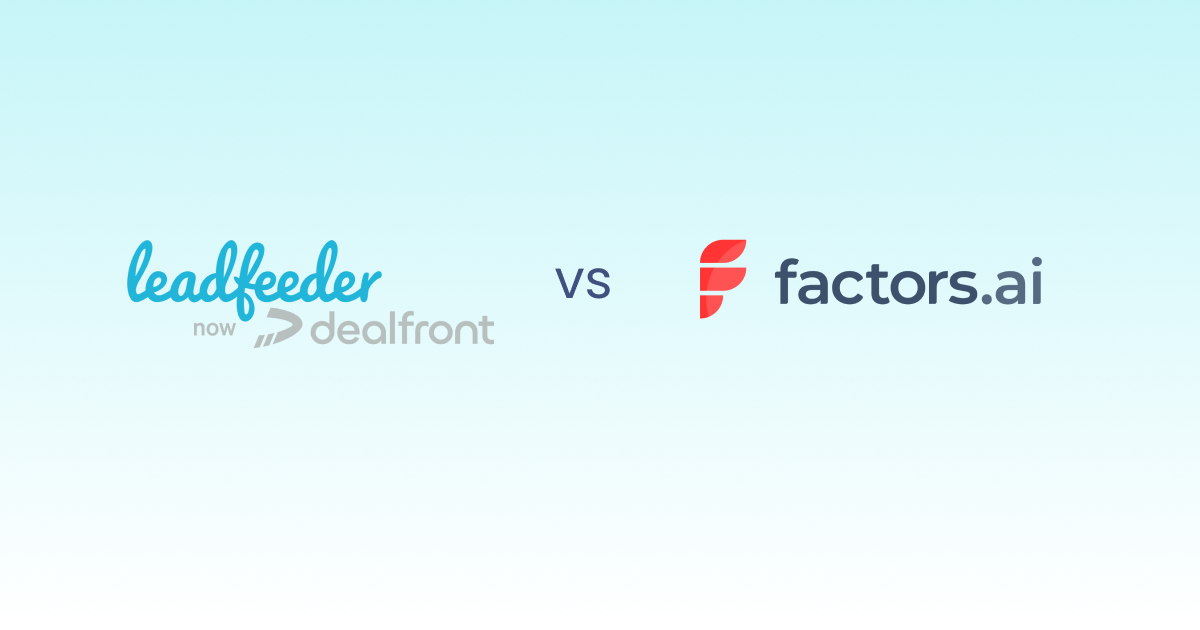
Leadfeeder [Dealfront] Vs. Factors: Compare Pricing & Features
Leadfeeder is a well-established B2B lead generation software that helps go-to-market teams identify and enrich anonymous website traffic. Leadfeeder has recently joined forces with another sales intelligence platform, Echobot, to form Dealfront — a Europe-centric B2B data platform.

As a result of this collaboration, Leadfeeder has consolidated multiple products, increased pricing plans, and limited the number of seats per account. Accordingly, several former-Leadfeeder users are considering switching to IP intelligence alternatives.
While Factors is a relatively new player in the account identification space, it’s quickly emerging as a popular alternative to Leadfeeder given its cost-effective plans and industry-leading match rates of up to 64%. That being said, every platform has its unique advantages and limitations that must be considered before making a purchase decision.
This blog compares Leadfeeder vs Factors to highlight features, benefits and drawbacks of each tool — and explore why one might make more sense for your use-cases over the other.
Leadfeeder vs. Factor.ai
Before diving into the differences between Leadfeeder and Factors, it’s worth highlighting a few commonalities between both tools given that they’re close alternatives to each other.
1. Account identification
Needless to say, identifying anonymous accounts on your website is a core functionality of both Leadfeeder and Factors. Both visitor identification tools use IP-based targeting technology to match anonymous traffic with company IPs so users can see who’s visiting their website without the need of form submissions or gated content.
This provides valuable insights into the nature of website traffic as well as which accounts to go after with retargeting ads or outbound outreach.
While both Leadfeeder and Factors rely on similar technologies, the accuracy with which they identify visitors can vary dramatically based on their respective databases. More on this later.
2. Account-level enrichment
Along with identifying company names, both Leadfeeder and Factors deliver robust account-level firmographic enrichment data such as industry, employee headcount, geography, revenue-range, and much, much more.
Account-level enrichment helps go-to-market teams quickly qualify whether a lead fits the target audience or ideal customer profile. This in turn helps focus marketing initiatives and sales outreach to a highly targeted set of accounts — as opposed to expensive and ineffective spray and pray tactics.
3. Implementation
Given that Leadfeeder and Factors employ similar IP-lookup technology to identify and track visitors, the implementation process is more or less identical. Both tools have quick and intuitive onboarding that involves the insertion of a light-weight code script onto the website. The script will soon start identifying visitors once activated.
Overall, the entire implementation process takes no more than 10min for either solution.
4. Real-time alerts
A really nifty feature offered by Leadfeeder and Factors is the ability for users to receive real-time Slack notifications when high-intent accounts are live on the website. These alerts can be configured to go off only when specific visitor criteria are met. For instance, teams can be notified only when a visitor is from a US-based SaaS platform with at least 100 employees and is on a high-intent page such as pricing.
Research suggests that contacting leads quickly dramatically improves chances of conversions. Accordingly, this is an especially valuable feature for sales reps looking to strike while the iron’s still hot.
5. Privacy-compliance
Leadfeeder and Factors are 100% privacy compliant. Both tools adhere to GDPR and CCPA privacy policies. Factors is also SOC2 type II certified. SOC2 information about Leadfeeder is not publicly available so we refrain from commenting on this.
With common features out of the way, let’s discuss what each visitor identification solution does better than the other.
What Factors does better than Leadfeeder
Here are a few reasons to consider Factors over other alternatives:
1. Data accuracy (outside Europe)
Rigorous comparative testing over a sample size of 20,000 domains reveals that Factors achieves industry-leading match rates of up to 64%.
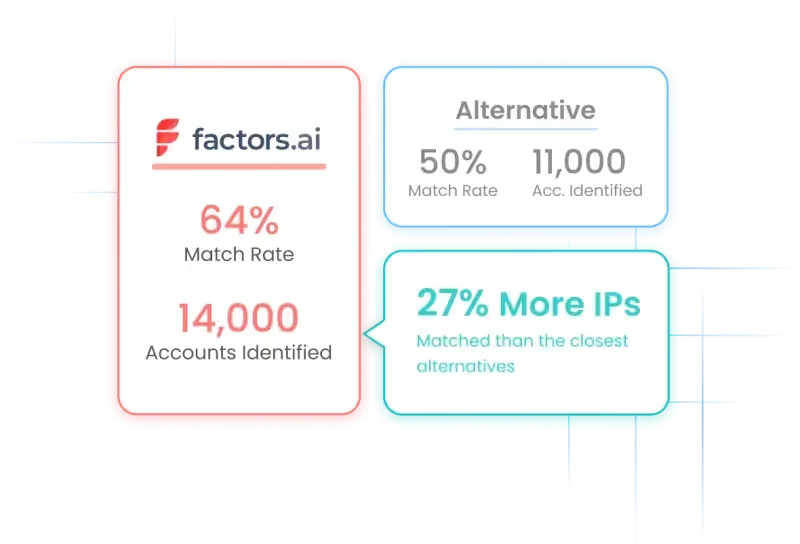
No doubt, both Leadfeeder and Factors boast impressive IP databases. In fact, Leadfeeder is at the forefront of IP-matching for Europe-specific data. Challenge with Leadfeeder may surface when targeting companies from US and other geographies outside Europe:
Leadfeeder records IPs for a whopping 26 millions companies in Europe but only about 8.5 million companies in the US and the rest of the world. Factors, on the other hand, covers almost double this figure with over 15 million companies.
Accordingly, if your business solely focused on the EU market, Leadfeeder is likely the better choice. But for global business, including the US, Factors should be the default. It taps into a relatively larger IP database to identify a wider range of companies from the rest of the world.
2. Account timelines
Account timelines is a feature unique to Factors that helps users visualize customer journeys and touchpoints in real time at an account and user level.
This is a powerful tool, especially in conjunction with visitor identification, to identify what influences different stakeholders within the same account to move from the awareness stage of the customer journey to closing the deal.
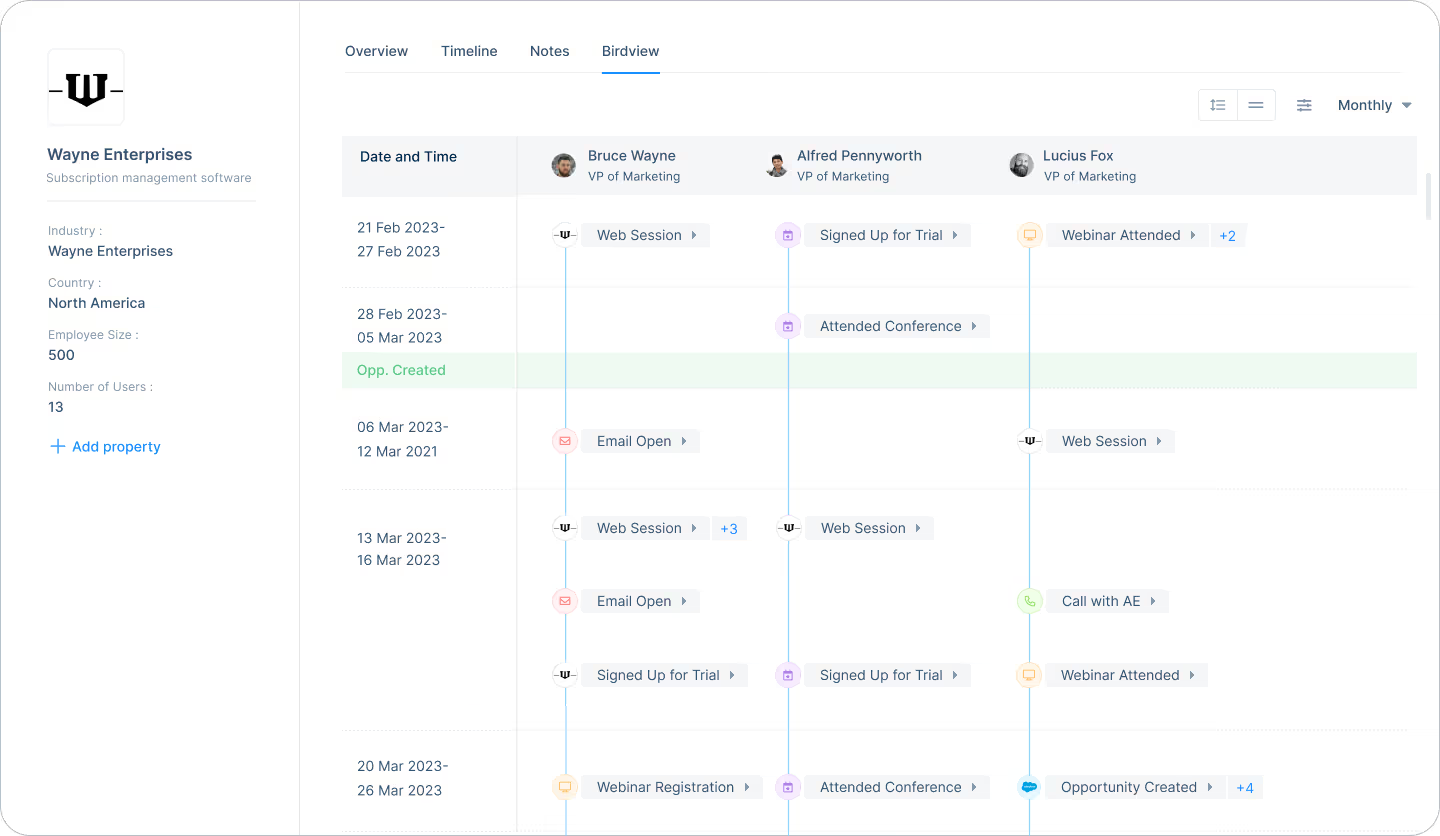
3. Advanced analytics
Factors is built upon strong analytics and attribution foundations. As a result, it’s capable of far more granularity in terms of reporting metrics and KPIs as compared to other marketing intelligence tools.
Factors auto-tracks website KPIs including:
- Page views
- Button clicks
- Percentage scroll depth
- Session duration
- Funnels (Eg: Homepage >> Pricing >> Blog >> Features)
- Custom KPIs and more
In addition to these out-of-the-box analytics, Factors integrated with ad platforms, website, CDPs, and CRMs to deliver powerful multi-touch attribution, path analysis, automated AI-fuelled insights, and more. In short, when it comes to analytics, Factors sheds light on what you need to know to drive more pipeline with less spend.

4. Customer success
While both Leadfeeder and Factors are relatively intuitive platforms, there’s no denying that they’re sophisticated products with at least some learning curves. With such products, it’s imperative to have onboarding support and dedicated customer success management — both of which Factors takes great pride in offering.

What Leadfeeder does better than Factors
Here are a few reasons to consider Leadfeeder over other alternatives:
1. Contact enrichment
While no solution can identify exactly who’s visiting your website at a user level, Leadfeeder natively provides users with a list of ideal prospects from the companies visiting a website.
For example, a fintech company may target the finance team from SaaS companies with over 10M in ARR. In this case, Leadfeeder can more or less find the contact info of CFO, VP finance, finance manager etc from companies already visiting your website — even though these professionals may not actually be the ones visiting.
This is useful for teams who’d rather not rely on specialized sales intelligence tools like Zoominfo or Apollo for dedicated contact-level data.
At the moment, Factors integrates with Apollo to provide this contact-level information. Since the majority of users rely on third-party service providers for contact information, this does not tend to be a blocker.
2. Integrations
Leadfeeder provides several native two-way integrations with popular sales and marketing tools including:
- Pipedrive
- Salesforce
- Activecampaign
- Mailchimp
- HubSpot
- Zapier
This helps users automatically upload account and contact information directly to their go-to-market tools and CRMs.
Factors integrates deeply with ad platforms, CRMs, CDPs, and more. While Factors does not provide native integrations with the full range of solutions Leadfeeder supports, it does integrate with Zapier/Make.com to support data pushback via Webhooks. At the moment, users can download csv files from Factors and upload them back into their preferred CRM, MAP, etc.
3. Native campaigns & promotions
Leadfeeder’s Promote feature allows users to target specific company IP-addresses with programmatic display ads across Google, Rubicon, Appnexus, and Pubmatic.
While the efficacy of display ads continues to be hotly debated, this may be a useful way to target companies that already visit your website and fit the ideal customer profile. The primary metrics to track these campaigns are impressions, CTR, and website visits.
Compare Leadfeeder Pricing
Leadfeeder is a relatively inexpensive solution as compared to established Leadfeeder alternatives such as Clearbit and Albacross. That being said, its recent collaboration with Echobot has revised pricing:
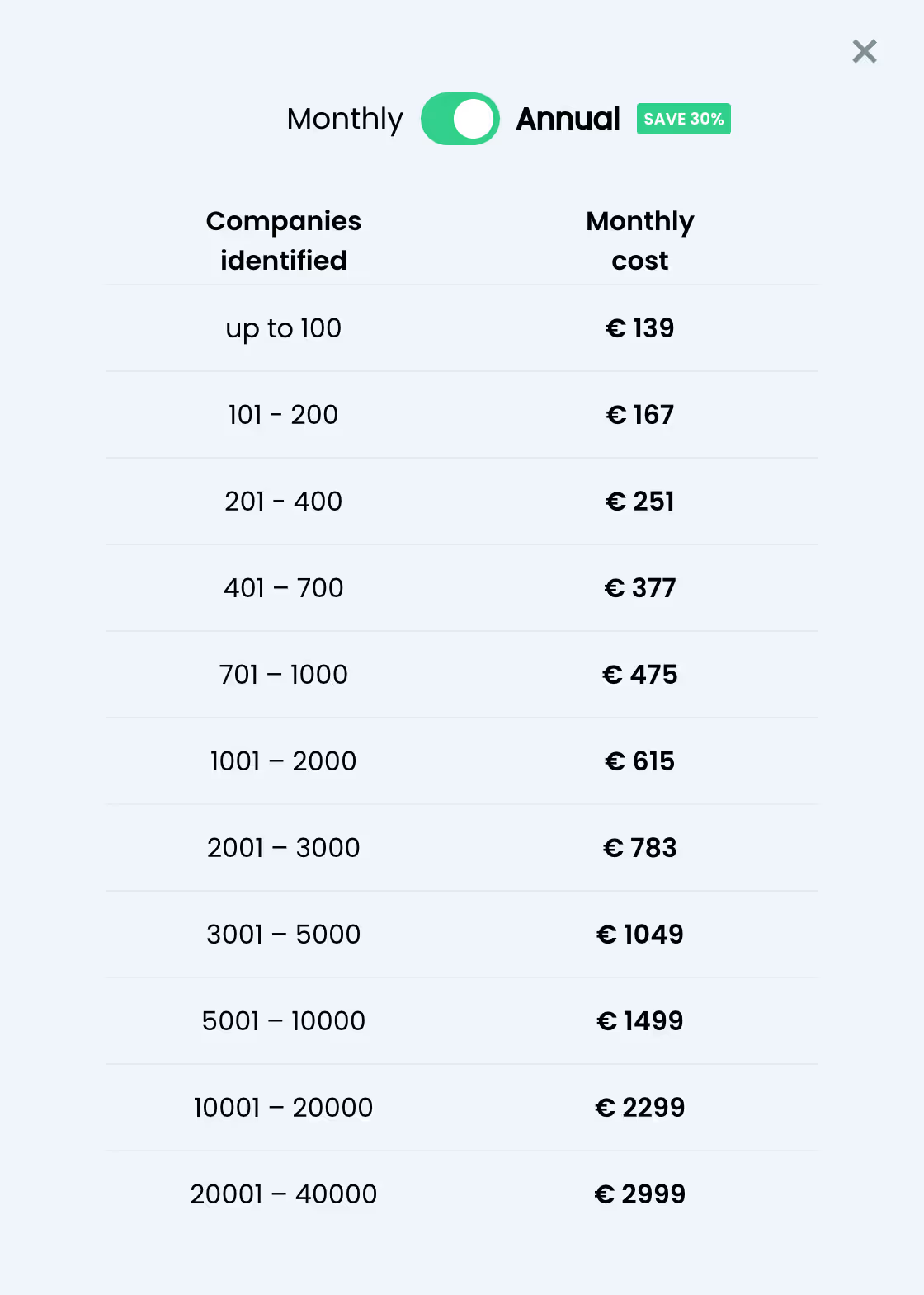
Now, Leadfeeder plans start at €139/mo ($152) to identify up to 100 accounts per month
Factors, on the other hand, continues to remain one of the most cost-effective marketing intelligence tools out there with plans starting as low as $99/month for up to 350 accounts identified and enriched per month. Factors pricing is based on the number of monthly website visitors and follows a gradient. Learn more about our pricing here: factors.ai/pricing
What’s the right tool for you?
This blog has compared Leadfeeder vs Factors.ai in terms of features, benefits, and drawbacks. Depending on your use-cases, one is likely to make a better fit for you than the other. Here are a couple of considerations:
- If data accuracy outside of Europe, cost-effectiveness, and holistic account scoring is a priority to you, Factors is likely better choice.
- If Europe-centric data and native contact enrichment is a priority to you, then Leadfeeder may be a better fit.
If you’d like to see how Factors achieves industry-leading match rates of up to 64%, book a demo here: See Factors in action

6 Tips to Align your Sales and Marketing Teams
Sales and marketing are like twins that don’t get along. Teams often confuse the two because they work along similar lines. In fact, both teams are often given a shared budget to divide amongst themselves.
In a study conducted by Harvard Business Review, when sales and marketing teams were asked to describe each, the two had nothing but negative comments. “Paper pusher” and “irrelevant” were the top terms used to describe the marketing team. On the other hand, some words that the marketing team used to describe the sales team included “incompetent” and “simple-minded” (Source).
Of course, this rift between sales and marketing has existed since the beginning of, well, sales and marketing.
But what if…what if…both sales and marketing could join forces to work together?
What can sales and marketing teams achieve when tightly aligned?
Well, organizations that have their sales and marketing teams on the same page enjoy the following:
- 36% higher customer retention rates
- 38% higher win rates (Source)
- 67% improvement in deal closures
- 209% more revenue from marketing (Source)
- 20% annual growth rate (Source)
- Save 30% Customer Acquisition Costs (Source)
Then the next question is—How can you get your sales and marketing teams on the same page? Here are a few tips that will help!
6 strategies to align sales and marketing
1. Align Goals to ROI
Sales and marketing teams have different ways of looking at their goals. While sales teams have monthly, quarterly, and annual deadlines, this concept is foreign to the marketing team that has never been linked to revenue performance.
Since both teams work in different styles, confining either one to follow the other process will result in unnecessary friction.
But the one thing that doesn’t change is their goals.
In the end, both their goals should be improving ROI. Taking the focus away from the journey and putting it on the result can even both the teams’ paces and unite them.
2. Employ Lead Qualification
Another area where both teams find themselves at odds is when a successful campaign from the marketing team does not translate into higher conversions for the sales team.

The culprit behind this is lead quality.
If the leads being generated are not interested in buying the company’s product, no matter how many prospects flow into your sales funnel, the end conversion rate will be the same.
One way to improve lead quality is by qualifying them.
Apart from this, lead qualification offers three other benefits: it help the marketing team understand whom they’re supposed to target, get both teams on the same page, and reduce the sales team’s efforts.
Then, how does one employ lead qualification?
One way to qualify a lead is to get both teams to sit together and build an ideal customer profile (ICP). An ICP is basically a customer profile of the business’s ideal buyers. It answers questions like how much they would be willing to spend, where they are more likely to live, what communication channels they are more likely to use, and what they are looking for.
The next step involves setting up a presales team that sorts the leads into invalid, uninterested, partly interested, and interested, before sending the leads forward. The invalid and uninterested leads get discarded.
The marketing team’s goal is to reduce the number of invalids and uninterested leads, whereas the sales team’s job is to convert the remaining two categories into clients.
These two steps can result in smoother operations between sales and marketing.
3. Use a CRM
Lack of communication is the biggest reason for disconnected sales and marketing teams. Since sales and marketing teams are usually swamped with their own work, it can get tricky to find time to interact with each other.
A Customer Relationship Management Software solves this problem by centralizing information. This allows different teams to access resources and monitor each other’s activities, keeping everyone on the same page.
CRMs also help nurture leads, track journeys, decrease duplication of efforts, provide deeper insights and analytics about customers, and score leads, all of which allow better decision-making and harmony between teams.
And if that isn’t enough, the software also provides sales and marketing automation, speeding up the groups’ efforts and saving them time.
4. Invest in Sales Enablement
Sales enablement is one of the most common methods companies employ to align their sales and marketing teams. How it helps companies is by:
1. Encouraging marketers and sales reps to interact
The marketing team needs to notify the sales team whenever they update new content. This forces them to reach out and interact with the sales reps. Similarly, sales reps must provide feedback on how the content helps them and what improvements can be made, thus, improving communication between the two.
2. Giving marketers and sellers an idea of what content resonates with customers the most
Once marketers create new content, they understand which content the sales team uses more and which is used less, thus helping them make better content to enable the reps.
Sales reps can also understand the type of prospects they are interacting with through the marketing team’s enablement content. This allows them to change their pitch according to the customer segment they are dealing with, which results in more closures.
3. Ensuring that the content remains on brand
When sellers have difficulty finding the right content, they might modify the material they already have to suit their sales purposes. This saves them time, but it might not necessarily be brand compliant.
Sales enablement allows representatives to modify materials on the fly using only pre-approved content. Furthermore, it gives marketers access to each modification made by each seller. This allows the marketing team to analyze what sellers are looking for in the material and which ones they are finding difficulty in navigating. This will be really beneficial for future asset optimization and improvement.
5. Attend Each Other’s Meetings
For companies that haven’t yet employed a CRM or an update tracking software, the best way for sales and marketers to keep tabs on each other’s updates is by actually attending their meetings.
Or better still, holding a dedicated joint meeting weekly or monthly can bring both teams on the same page. This ensures everyone is on the same page regarding that quarter's goals, tactics, expectations, strategies, and performance progress.
Here are some tips for holding a fruitful sales and marketing meeting
1. Set a proper agenda
Collect the agendas to be discussed beforehand and allot specific times for them to ensure all the topics are discussed. Remember to keep buffer time in case the topics drag on and the time taken to finalize a decision on that agenda.
2. Keep changing the themes
One way to avoid having complicated agendas with broad topics is by grouping them under different themes. Keeping one theme per weekly meeting can simplify the agenda and burden both teams less.
Some popular sales and marketing themes include:
- Strategies Discussion – Held to discuss the strategies that have been working and the strategies that need to be changed or dropped.
- Performance Reviews – How the teams’ efforts contribute to achieving goals and how much they have progressed.
- Buyer Behaviour – Held to discuss any changes and improvements that can be made regarding buyer engagement or to discuss the results of an already published campaign.
- CCC review – Held to review and discuss marketing and sales content, campaigns to be published or made, and any notable moves competitors have made.
And since there is a theme for each meeting, all members of both teams need not have to attend. Instead, they can look at the meeting minutes later, saving them time to do other work.
3. Share the agenda beforehand and assign representatives to speak
The last way to ensure fruitful meetings is to share the topics to be discussed beforehand. This gives both teams time to prepare in advance. Furthermore, assigning representatives saves time for reps and marketers who must sit through the entire length of the meeting to discuss minute topics.
6. Talk out the discrepancies
It’s common to have disagreements between sales reps and marketers over different issues. However, while they might seem trivial, these conflicts must be dealt with utmost care to not give the illusion that one team is favored over the other.
Another common issue is that both teams might not say out loud whether they agree or not with each other for the fear of retaliation or confrontation.
One solution to these problems is encouraging both teams to provide constructive criticism. Sales and marketing employees can be taught how to provide feedback without sounding rude.
And to ensure that the feedback is formalized and the interactions stay respectful, it can be organized on dedicated venues like forums or a weekly critics session.
The final step is to rinse and repeat these 6 steps. But remember, like all other strategies, they will soon become obsolete and ineffective if your business doesn’t regularly revisit and update them.
Ultimately, aligning both sales and marketing teams is always important, no matter how big or small a business is. While these are very effective strategies, each company knows its employees the best and, thus, should also consider additional custom methods apart from these as well.
